by Scott Muniz | Jun 26, 2020 | Uncategorized
This article is contributed. See the original author and article here.
It’s been a year since Microsoft acquired Citus Data, and while there have been strange changes such as my team now officially being called “Ninjas”, we still often get unusual and interesting Postgres “puzzles” to solve. If you’re not familiar, Citus is an open source Postgres extension that scales out Postgres horizontally that is now available as Hyperscale (Citus) as part of our managed Postgres service on Azure.
Recently, our friends at ConvertFlow, a long-time Citus customer approached our team with a particularly interesting Postgres challenge. ConvertFlow has what we call a “HTAP” workload, featuring an application that gives marketers a personalized way to guide website visitors to become leads, customers, and repeat buyers. For those of you that are curious, HTAP means that they have a mixed multi-tenant SaaS and also real-time analytics workload multi-tenant SaaS.
The problem: 2 billion rows & ongoing growth meant ConvertFlow would soon face an integer overflow issue
ConvertFlow has a pair of Postgres tracking tables sharded across multiple servers in a Citus database cluster at the heart of their application. A good chunk of their SaaS application workflow either updates or reads from these Postgres tables. ConvertFlow’s usage has shot up over the past few years, so as you can imagine these tables got really big over time. Today, there are two billion rows across the two tracking tables, those tables alone get 40,000 reads a minute, and the number of reads is climbing.
This type of growth, unfortunately, often leads to a problem: integer overflow.
Each of ConvertFlow’s Postgres tracking tables had a bit over 1 billion rows, and each table used an integer primary key that was set to autoincrement. This meant that each table had a row with an integer that was increasing with every row added. Unfortunately, Postgres limits the maximum size of the integer type to 2,147,483,647. This meant that the primary key columns were already roughly half full, and with ConvertFlow’s continued growth, integer overflow would soon become an issue.
Fortunately, Postgres has another numeric data type called bigint, with a limit of 9,223,372,036,854,775,807, or roughly 9 quintillion. While Citus database clusters can get extremely large, we’ve never encountered anything close to hitting the bigint limit.
This realization led us to our solution: if we switch from the integer data type to bigint in our customer’s Postgres tables, we’d be all set. Sounds easy, right?
Numeric Types available in Postgres
From the PostgreSQL documentation chapter on Data Types, the table of built-in Numeric Types
Numeric Types available in Postgres
More challenges: aggressive Postgres locking might cause too much downtime
Well, one minor detail got in the way here: Postgres locks when changing the type of a column. Specifically, Postgres gets an ACCESS EXCLUSIVE lock, a very aggressive type of lock, when changing types. In Postgres, ACCESS EXCLUSIVE locks prevent updates, inserts, and even reads from going through for the duration of the update. As a result, for all practical purposes changing the Postgres data type would take the application down.
Some quick tests showed that there would be approximately 10 hours of downtime for the change to persist across 2 billion rows. While this is relatively quick given the amount of data, 10 hours was definitely way too long of a maintenance window. So, we devised a plan to address this concern.
The plan: make new BIGINT columns, copy the data to them, and switch over later
The plan started out simply enough. We’d make a new BIGINT column in each of the Postgres tables in the Citus cluster, mirror the data from the existing columns to the new ones, and switch over the application to use the new columns.
This seemed to be a great idea—we could set up a Postgres trigger for new or updated values and slowly do batch updates to copy over the existing values as they came in. To make it even better, we could schedule the batch updates using the open source pg_cron extension, which let us do those batch updates overnight without having to stay up late.
The only maintenance window would occur when we later switched over to using our new Postgres bigint columns. It would definitely be a bit slower, but we’d also have far less locking. In addition, we could avoid periods of high activity by doing batch updates of existing rows. We still had a few months of runway left before we ran out of usable integers, so the time to finish wasn’t an urgent factor yet.
The twist: how to maintain existence of primary keys at all times?
We quickly realized that we’d overlooked a surprising twist. The integer columns were part of the primary keys on the tables in question, and ConvertFlow uses a Rails app, so we had to maintain the existence of primary keys at all times to keep the app happy.
We could drop the primary key in a transaction and make a new one, but with 2 billion rows in the distributed Postgres tables, that was still likely to take an hour or two, and we wanted a shorter maintenance window.
The fix: Postgres’s wonderful ADD table_constraint_using_index
Fortunately, Postgres has another cool feature we could use in this case: ADD table_constraint_using_index
. You can use this argument when creating a constraint to promote a unique index to a primary key, which lets Postgres know that the unique constraint is valid. That means that Postgres doesn’t need to do a full table scan to check validity of the primary key. As a result, we could drop the existing primary key and very quickly make a new one using that BIGINT column. While this would still involve a brief lock for the ALTER TABLE
to complete, this approach would be much faster. We discussed the idea with ConvertFlow, wrote some Rails migrations, tested everything, and then scheduled a maintenance window.
With everything now figured out, we started. We ended up doing everything in a Rails migration instead of raw SQL, but in the interest of making this more generally applicable, here’s the pure SQL version of what we ran:
-- First, make basic modifications to get the new column added. We've got a composite primary key, so the new constraint has 2 columns in it to match the original.
ALTER TABLE target_table ADD COLUMN column_bigint BIGINT;
ALTER TABLE target_table ADD CONSTRAINT future_primary_key UNIQUE (column_bigint, other_column);
-- Next, ensure that all incoming updates and inserts populate that column:
CREATE OR REPLACE FUNCTION populate_bigint
RETURNS trigger AS
$BODY$
UPDATE target_table SET new.column_bigint=new.original_column;
$BODY$;
CREATE TRIGGER populate_bigint_trigger BEFORE INSERT OR UPDATE ON target_table
FOR EACH ROW
EXECUTE populate_bigint();
-- Now, populate the column for all existing rows. We’re doing this in chunks so we can pause and resume more easily.
UPDATE target_table SET column_bigint = original_column WHERE column_bigint IS NULL LIMIT 10000;
--Repeat last line as needed. We scheduled this using pg_cron so we didn't have to keep running different commands.
-- Now that everything’s caught up, take a few minutes of downtime, and switch over. We’re doing this in a transaction, just in case.
BEGIN;
ALTER TABLE target_table DROP CONSTRAINT original_pkey;
ALTER TABLE target_table ADD CONSTRAINT new_pkey PRIMARY KEY USING INDEX future_primary_key;
END;
tl;dr: Taking it slow meant only 20 minutes of downtime for 2 billion rows.
We ran this, and it worked! It ended up requiring about 20 minutes of downtime, most of which was waiting for long-running transactions to finish and doing testing to make sure we got it all right. This fit very nicely into the maintenance window the ConvertFlow team had planned. What a fantastic improvement over the 10+ hours we had originally estimated, and at every single point in the process we had an easy way to back out if we had missed any details. Success!
If you’re at the database size where integer overflow is a problem for you, it’s probably worth
checking out Citus as well. These Postgres tips work fine with and without the Citus extension, but it never hurts to be ready to scale out.
by Scott Muniz | Jun 26, 2020 | Uncategorized
This article is contributed. See the original author and article here.
Writers today, both students and working professionals, have an incredible array of reference material available. It can be confusing to know when content requires attribution and how to cite it appropriately – these are learned skills that can be time-consuming to teach and difficult to remember.
From discussions with students and teachers, we know that writing tools today do not alert students to the need for a citation or help them add citations early enough in the writing process.
“There have been a lot of changes lately in how we teach plagiarism and make it more of a learning process rather than a gotcha. The fact that this can catch things as students are writing is excellent.” – High School Teacher, History
We are pleased to announce that Editor’s Similarity checker feature, available in Microsoft Word for Microsoft 365 EDU A3 and A5 customers, is currently available in Office preview builds. The feature will release to general availability in July.
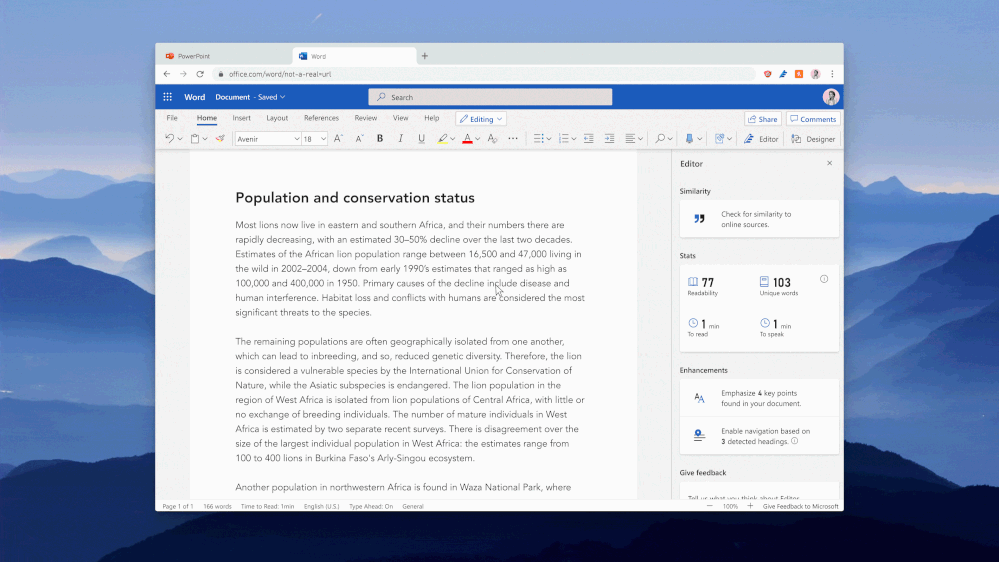
Powered by Bing Search, the Similarity checker can identify and help writers with originality in their writing and learn more about appropriate attribution through tools that facilitate the easy insertion of relevant citations. This can aid writers in focusing less on the mechanics of writing, and more on the content.
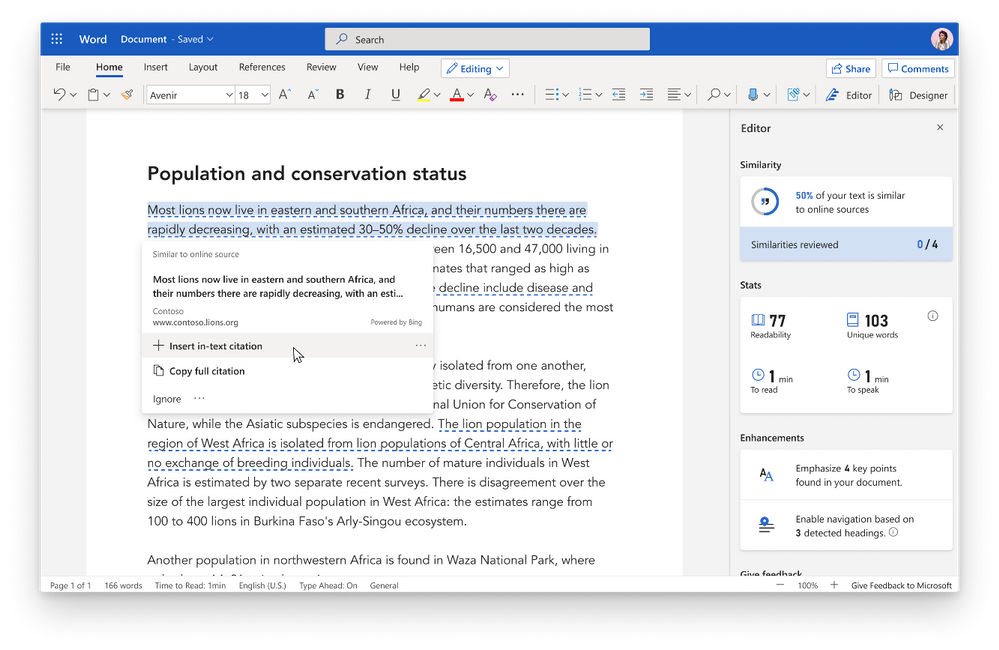
Critically, the Similarity checker makes this functionality available to students while they are still in the writing process. While in the past teachers viewed plagiarism-checking software as a punitive/”gotcha” moment at submission time, teachers are trending towards empowering their students to find their authentic voice while leveraging citations as appropriate. Coupling similarity detection with citation tools presents an opportunity to provide guidance when it will be most effective to improve writing outcomes.
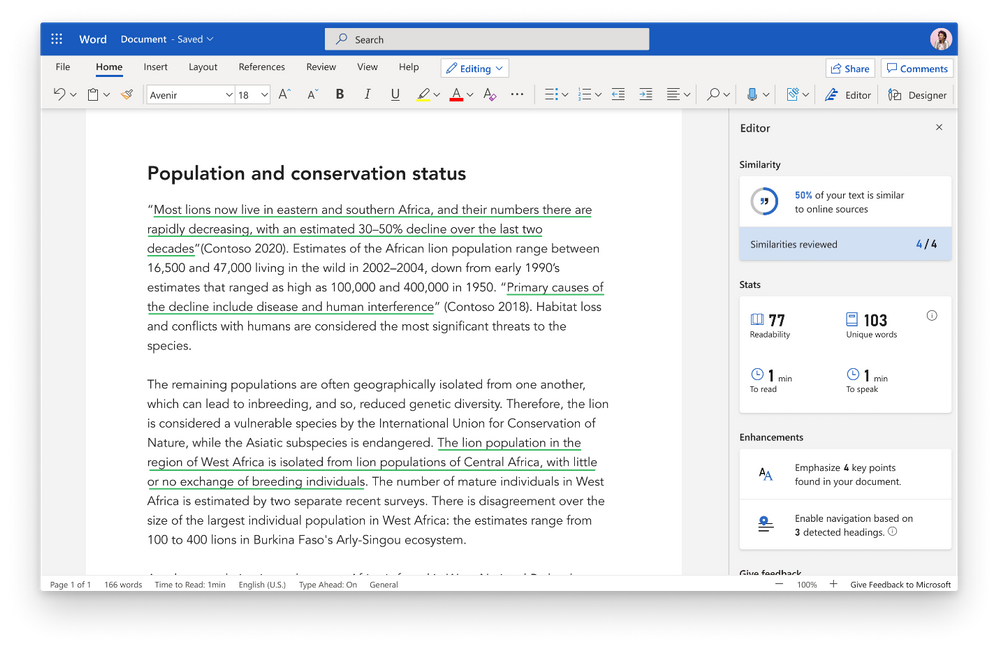
“At the end of the day, no teacher wants to fail a student for plagiarizing. I think of it as my own failure when a student fails. Most teachers would feel glad to know that students have a bit more empowerment.” – High School Teacher, English
To learn more about Editor’s Similarity checker in Microsoft Word, visit http://aka.ms/similaritychecker.
To learn more about Microsoft Editor, visit https://www.microsoft.com/en-us/microsoft-365/microsoft-editor.
Michael Heyns
Microsoft Word Program Manager
by Scott Muniz | Jun 26, 2020 | Uncategorized
This article is contributed. See the original author and article here.
So many good videos this week, it was hard to pick three! But between Excel On Fire bringing his new hat to the table, OfficeNewb running a live Q&A, and Leila Gharani waving us over to the Data tab, we think we found some fun highlights. If you find these helpful, be sure to check out their channels!
Learn more about the Microsoft Creators Program.
See more from Excel On Fire
See more from OfficeNewb.com
See more from Leila Gharani
by Scott Muniz | Jun 26, 2020 | Azure, Microsoft, Technology, Uncategorized
This article is contributed. See the original author and article here.
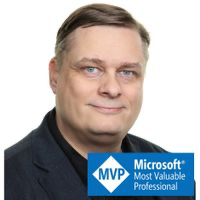
Why to OneNote instead of Whiteboard?
Vesa Nopanen is a Principal Consultant in Office 365 and Modern Work, and is passionate about Microsoft Teams. He enjoys helping and coaching customers to find benefits and value when adopting new tools, methods, ways of working and practices for a daily work-life equation. He focuses especially on Microsoft Teams and how it can change how organizations work. He lives in Turku, Finland. Follow him on Twitter @vesanopanen.
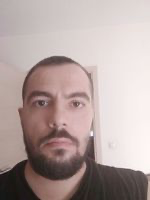
Azure SQL – Failover Group Configuration
George Chrysovalantis Grammatikos is based in Greece and is working for Tisski ltd. as an Azure Cloud Architect. He has more than 10 years’ experience in different technologies like BI & SQL Server Professional level solutions, Azure technologies, networking, security etc. He writes technical blogs for his blog “cloudopszone.com“, Wiki TechNet articles and also participates in discussions on TechNet and other technical blogs. Follow him on Twitter @gxgrammatikos.
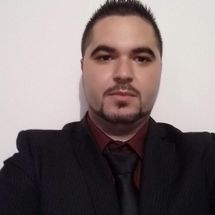
Why model binding to JObject from a request doesn’t work anymore in ASP.NET Core 3.1 and what’s the alternative?
Anthony Giretti is a specialist in web technologies with 14 years of experience. He specializes in particular in Microsoft .NET and he is currently learning the Cloud Azure platform. He has twice received the Microsoft MVP award and he is also a certified Microsoft MCSD and Azure Fundamentals. Follow him on Twitter @anthonygiretti.
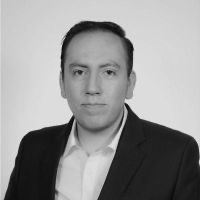
Build, Run, Deploy Docker Container to Azure Container Registry and deploy it as Web App for Containers
Dave Rendón has been a Microsoft Azure MVP for 6 consecutive years. As an IT professional with more than 10 years of experience, he has a strong focus on Microsoft technologies and moreover on Azure since 2010. He supports the business developers and sales teams at Kemp from a technical level. I also support the account managers by developing a firm understanding of their customer’s technical dilemma(s) and providing a sound technical solution. Follow him on Twitter: @DaveRndn
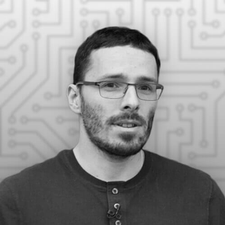
Entity Framework Core’s ultimate escape hatch
Jiří Činčura is an independent developer focusing on data and business layers, language constructs, parallelism and databases. Specifically Entity Framework, asynchronous and parallel programming, cloud and Azure. He’s a Microsoft MVP and you can read his articles, guides, tips and tricks at www.tabsoverspaces.com. Follow him on Twitter @cincura_net.
by Scott Muniz | Jun 26, 2020 | Uncategorized
This article is contributed. See the original author and article here.
Azure Synapse Analytics Workspace enables you to read the files from Azure Data Lake storage using OPENROWSET(BULK <file url>). In this article you will see how to grant minimal permission to the users who need to analyze files with OPENROWSET(BULK) function.
When you try Azure Synapse workspace, you usually start with full permissions (for example as database owner). Once you need to let other users access the files on Data Lake, you probably think that is the minimal permission that you need to assign to user to let them just do ad-hoc analysis without any other permissions.
In this post you will learn how to configure minimal permission to needed to analyze the files on Azure storage.
Creating server principals
SQL endpoint in Synapse workspace uses standard T-SQL syntax to create principals who can access your data. The following statement creates a new login with username testprincipal and password VeryStrongAndSecurePassword1234!!!:
create login testprincipal
with password = 'VeryStrongAndSecurePassword1234!!!';
Using this T-SQL statement you can create new principals that are assigned to the public role.
Let’s see what we can do with this principal. If we login using this username/password we can try to run the following queries:
select name from sys.databases
-- success
use SampleDB
--Msg 916, Level 14, State 2, Line 1
--The server principal "testprincipal" is not able to access the database "SampleDB" under the current security context.
select top 10 *
from openrowset(bulk 'https://pandemicdatalake.blob.core.windows.net/public/curated/covid-19/ecdc_cases/latest/ecdc_cases.parquet',
format='parquet') as a
-- Success
By default, a new user can see all databases, execute OPENROWSET to query files on Azure Data Lake storage, but cannot access other databases or create objects. This can be verified using the following function:
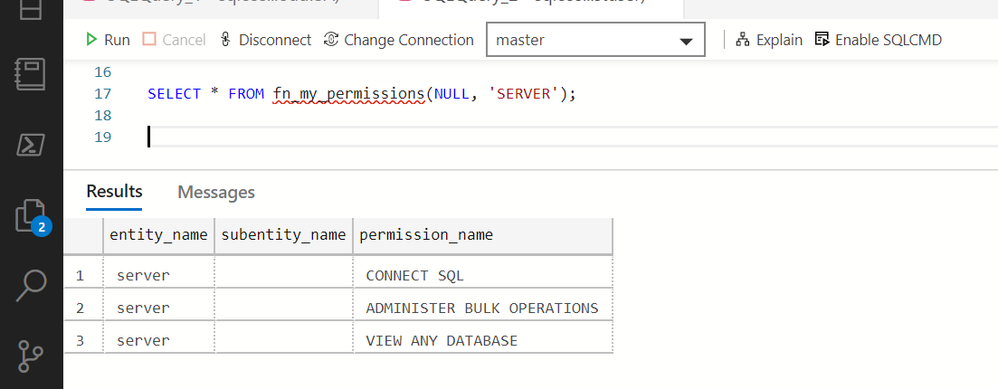
New login is in the public role and has the permissions to view any database and to run ad-hoc query using OPENROWSET, but no other permissions.
This version of OPENROWSET enables principals to public access storage or in case of Azure AD principal to access files on the storage where storage admin granted Storage Blob Data Reader RBAC role to Azure AD user who access. If you need more fine grained impersonation mechanism, you should create data sources and protect them with credentials in databases. the following section will describe how to enable principal access objects in databases.
Configuring database permissions
Let’s enable user to access database. In the context of some database such as SampleDB execute the following statement:
create user testprincipal for login testprincipal;
This statement created a database user that will access current database using the login defined in the previous script. This principal can now connect to SampleDB database, can still use OPENROWSET with absolute URL to read files from storage, and it can also use OPENROWSET that references some public data source:
SELECT TOP 10 *
FROM
OPENROWSET(
BULK 'puYear=*/puMonth=*/*.parquet',
-- this is a data source that referneces a public location (without credential)
DATA_SOURCE = 'YellowTaxi',
FORMAT='PARQUET'
) AS nyc
In this case, data source doesn’t have credential and references public location:
CREATE EXTERNAL DATA SOURCE YellowTaxi
WITH ( LOCATION = 'https://azureopendatastorage.blob.core.windows.net/nyctlc/yellow/')
However, this principal cannot read some files using a pre-defined data source that is protected with credential:
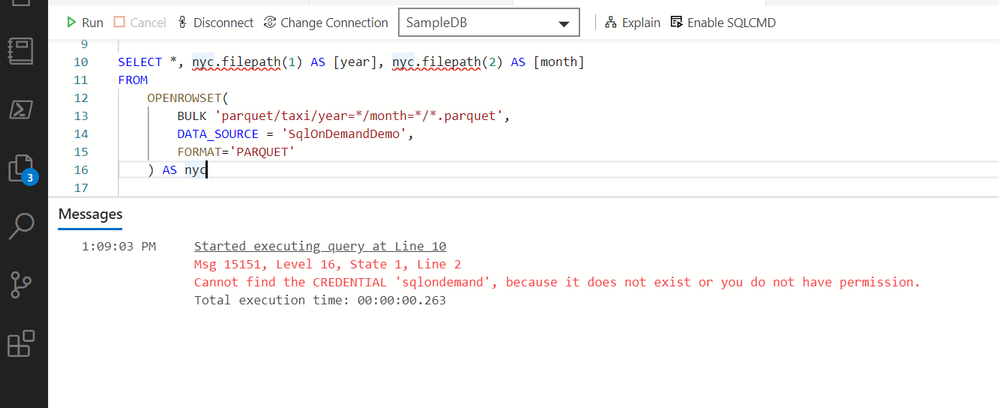
The issue here is that data source uses credential to access storage, and the current user cannot reference this credential:
CREATE EXTERNAL DATA SOURCE SqlOnDemandDemo WITH (
LOCATION = 'https://sqlondemandstorage.blob.core.windows.net',
CREDENTIAL = sqlondemand
);
In order to access the files via crednetial-protected data source, the principal needs to have references permission on the underlying database scoped credential (in this case sqlondemand credential) that is used in data source:
grant references
on database scoped credential::sqlondemand
to testprincipal;
Note that the user who uses OPENROWSET with data source still need to have ADMINISTER BULK OPERATIONS permission. If you deny this permission, the principal cannot use OPENROWSET anymore:
-- execute from master database context:
deny ADMINISTER BULK OPERATIONS to testprincipal;
Note that you need to explicitly DENY permissions and you cannot just REVOKE this permission like in this example:
revoke ADMINISTER BULK OPERATIONS to testprincipal;
If you try to revoke this permission testprincipal will still be able to execute OPENROWSET (you can confirm this using fn_my_permissions(NULL, ‘SERVER’) function)
This might confuse you, but the reason is that ADMINISTER BULK OPERATIONS is not initially granted to testprincipal. testprincipal belongs to the public role, and in synapse SQL endpoint, public role has ADMINISTER BULK OPERATIONS permission by default. If you want to revoke this permission, you would need to revoke it from the public role using this statement:
revoke ADMINISTER BULK OPERATIONS to public;
If you will have just the users who will analyze files in ad-hoc manner, you can leave this permission to public role. Otherwise it might be good to revoke it and create separate data analyst role that will have this permission.
Recent Comments