by Scott Muniz | Jun 29, 2020 | Azure, Microsoft, Technology, Uncategorized
This article is contributed. See the original author and article here.
Karishma Dixit – Microsoft Threat Intelligence Centre
Many audit logs contain multiple entries that can be thought of as a sequence of related activities, or session. In this blog, we use a Jupyter notebook to hunt for anomalous sessions in data ingested into Azure Sentinel. We use OfficeActivity logs to illustrate this in detail, though a similar approach can be applied to many other log types. A session is a timebound sequence of activities linked to the same user or entity. We consider an anomalous session one that has sequences (of events or activities) that we don’t see in other user sessions.
We demonstrate how to sessionize, model and visualise the using msticpy and take a deep dive into the underlying implementation of the modelling methodology. We focus our analysis on sessions produced by users interacting with the Office 365 PowerShell API. This will provide insight into possible malicious activity in Office 365 Exchange and Sharepoint.
Office 365 PowerShell API is a management API which can be used to manage Office365 services remotely via PowerShell Cmdlets. As well as providing users and administrators with convenient access to management functions of Office365, this API is also an attractive target for attackers as it provides many features that can be abused for persistence and data exfiltration. Some examples of potentially malicious commands are:
-
- Kohn” –DeliverToMailboxAndForward $true – ForwardingSMTPAddress “badguy@bad.com”
- This example delivers Douglas Kohn’s email messages to Douglas’s mailbox and forwards them to badguy@bad.com’s mailbox
- Collection
- New-MailboxSearch –Name “my search” –SourceMailboxes “Finance” –SearchQuery ‘Subject: “Your bank statement”’
- This example searches through the mailboxes of the “Finance” distribution group for emails which have the subject “Your bank statement”
- Permission changes
- New-ManagementRoleAssignment
- This cmdlet could be used by an attacker for privilege escalation
Since the Exchange Online cmdlets give us some good attack examples, we choose to focus our analysis on this subset of the API cmdlets. However, this is only a subset of what is available in the Office logs.
Using Jupyter Notebooks
Jupyter notebooks are a nice way of running custom python code on data from your Azure Sentinel workspace. If you are new to Jupyter notebooks and would like to understand how it can help with threat hunting in Azure Sentinel, Ian Hellen wrote a series of blogs covering the topic.
We recently checked in a new notebook to the Azure Sentinel Notebooks GitHub repository. This notebook enables the user to sessionize, model and visualise their Exchange data from their Azure Sentinel OfficeActivity logs. It also acts as an example by which you can extend to investigate other security log types. It achieves this by making use of the new anomalous_sequence subpackage from msticpy. For details on how this library works, please read the docs and/or refer to this more documentation heavy notebook.
Below we go into more detail on how this notebook makes use of the anomalous_sequence subpackage to analyse Office management activity sessions.
Creating Sessions from your data
First, we need to sessionize the data. We define a session to be an ordered sequence of events that are usually linked by a common attribute (e.g. user account). In this blog, we treat the Office Exchange PowerShell cmdlets as the events.
The anomalous_sequence subpackage can handle three different formats for each of the sessions:
- Sequence of just events
[“Set-User”, “Set-Mailbox”]
- Sequence of events with the accompanying parameters used
[
Cmd(name=”Set-User”, params={“Identity”, “Force”}),
Cmd(name=”Set-Mailbox”, params={“Identity”, “AuditEnabled”})
]
- Sequence of events with the accompanying parameters and their corresponding values.
[
Cmd(name=”Set-User”, params={“Identity”: “test@example.com”, “Force”: “true”}),
Cmd(name=”Set-Mailbox”, params={“Identity”: “test@example.com”, “AuditEnabled”: “false”})
]
This allows you to build your analysis using only the event/cmdlet name, the event plus the parameter names, or the event plus the parameter names and their accompanying values.
To create sessions from timestamped events, we define the notion of –related variables. A user-related variable is a data value that maps an event to a particular entity (e.g. UserId, ClientIP, ComputerId). This allows us to group events belonging to a single entity into sessions. We sort the events by both the user related variables and timestamp in ascending order. Then each time one of the user related variables changes in value, a new session would be created. For the Office Exchange use case, we set the user related variables to be “UserId” and “ClientIP”.
We also impose the following time constraints: each session can be no longer than 20 minutes in total, and each event in a session can be no more than 2 minutes apart. The time constraints used here are somewhat arbitrary and can be adjusted for different datasets/use cases.
We do the sessionizing directly inside of our KQL query to retrieve data from Azure Sentinel. In order to achieve this, we make use of the row_window_session function.
However, if your data is stored somewhere else and you wish to do the sessionizing using Python, then you can use the sessionize_data function from msticpy.
Here is the KQL query we use to both retrieve and sessionize the office exchange data:
let time_back = 60d;
OfficeActivity
| where TimeGenerated >= ago(time_back)
// filter to the event type of interest
| where RecordType == 'ExchangeAdmin'
// exclude some known automated users (optional)
| where UserId !startswith "NT AUTHORITY"
| where UserId !contains "prod.outlook.com"
// create new dynamic variable with the command as the key, and the parameters as the values (optional – only if you want to include params in the model)
| extend params = todynamic(strcat('{"', Operation, '" : ', tostring(Parameters), '}'))
| project TimeGenerated, UserId, ClientIP, Operation, params
// sort by the user related columns and the timestamp column in ascending order
| sort by UserId asc, ClientIP asc, TimeGenerated asc
// calculate the start time of each session into the "begin" variable
// With each session max 20 mins in length with each event at most 2 mins apart.
// A new session is created each time one of the user related columns change.
| extend begin = row_window_session(TimeGenerated, 20m, 2m, UserId != prev(UserId) or ClientIP != prev(ClientIP))
// summarize the operations and the params by the user related variables and the "begin" variable
| summarize cmds=makelist(Operation), end=max(TimeGenerated), nCmds=count(), nDistinctCmds=dcount(Operation),
params=makelist(params) by UserId, ClientIP, begin
//optionally specify an order to the final columns
| project UserId, ClientIP, nCmds, nDistinctCmds, begin, end, duration=end-begin, cmds, params
// filter out sessions which contain only one event (optional, commented out in this case)
//| where nCmds > 1
Once you have run this KQL query, you will end up with 1 row per session. Something like this:
Example output of the KQL query
Let’s see what needs to be done to this dataframe before we can start the modelling. The “cmds” column is already in an allowed format (list of strings). However if we would like to include the accompanying parameters for each of the cmdlets (and the values set for those parameters) in the modelling stage, then we need to make sure the “params” column is a list of the Cmd datatype. We can see that the “params” column is a list of dictionaries which is not quite what we want. Therefore, we must apply a small pre-processing step to convert it. The details for this pre-processing step can be found in the anomalous_sequences subpackage documentation.
Example of the pre-processed dataframe
and scoring the sessions
Now that we have created the sessions from our Office Exchange logs, it is time to train a model on them.
Since we have chosen an unsupervised approach, we do not require our sessions to have labelled outcomes (1 for malicious, 0 for benign). We train a model by estimating a series of probabilities for the cmdlets and optionally for the accompanying parameters and values if provided. We then use these estimated probabilities to compute a “likelihood score” for each session. This allows us to rank all our sessions in order from least likely to most likely. We can then hunt for anomalous activity by focusing on the sessions with lower likelihoods scores.
To calculate the likelihood scores for the sessions, we use a sliding window approach. We do this by fixing a window length, of size 3 for example. The for a session is then calculated by computing the likelihood of each window of length 3, and then taking the lowest likelihood as the score.
More details about the modelling are provided later in the deep dive section.
We wrote a high-level function in msticpy which takes a pandas dataframe as input, trains the model and then assigns a likelihood score to each session. The output is a copy of the input dataframe with additional columns appended for the likelihood score and the rarest window in the session. The lower likelihood scores correspond with the more rare/anomalous sessions.
Assuming your dataframe from the sessionizing section is called “data” and has the sessions contained in column “param_value_session”, you can run this snippet:
from msticpy.analysis.anomalous_sequence import anomalous
modelled_df = anomalous.score_sessions(
data=data,
session_column='param_value_session',
window_length=3
)
This function will infer what type of sessions you have provided and will do the modelling accordingly. If your sessions are just a list of the cmdlets, then it will model just the cmdlets. If instead they are a list of the Cmd datatype, then it will include the parameters (and values if provided) in the modelling.
You can then sort the resulting dataframe in ascending order of the likelihood score (scores close to zero are least likely/more anomalous) to see which sessions have been deemed the most anomalous by the model:
modelled_df.sort_values('rarest_window3_likelihood').head()
Alternatively, if you wanted to return all sessions within a certain threshold (on the likelihood score), you could run the following:
modelled_df.loc[modelled_df.rarest_window3_likelihood < 0.001]
For more control over the model configuration, Model class directly. This will allow you to choose whether start and end tokens are used, whether the geometric mean is used and will provide access to some additional useful methods.
Notice that so far, we have trained a model on some data and then used the trained model to assign a likelihood score to each of the sessions from the same dataset. However, another use-case could be to train the model on a big batch of historical data and then use the trained model to compute likelihood scores for new sessions (not present in the training data) as they arise. We do not currently have a high-level implementation for this use case. However it is still possible via usage of the rarest_window_session functions from the anomalous_sequence utility functions. We hope to include a high-level implementation for this use case in a future release of msticpy.
Visualising your modelled sessions
We now demonstrate how you can visualise your sessions once they have been scored by the model.
We do this using the visualise_scored_sessions function from msticpy.
- The time of the session will be on the x-axis
- The computed likelihood score will be on the y-axis
- Lower likelihoods correspond with rarer sessions
# visualise the scored sessions in an interactive timeline plot.
anomalous.visualise_scored_sessions(
data_with_scores=modelled_df,
time_column='begin', # this will appear on the x-axis
score_column='rarest_window3_likelihood', # this will appear on the y axis
window_column='rarest_window3', # this will represent the session in the tool-tips
source_columns=['UserId', 'ClientIP'] # specify any additional columns to appear in the tool-tips
)
This function returns an interactive timeline plot which allows you to zoom into different sections and hover over individual sessions Because the likelihood score is on the y-axis, the more rare/anomalous sessions will be towards the bottom of the chart. can be useful for spotting patterns over time. For example, it could help to rule out some series of benign scheduled sessions when you are trying to hunt for malicious sessions and see if there are any temporal patterns associated with the anomalous activity.
Please note that the example plot provided here is based on synthetic data from one of our and is therefore not representative of what your own data will look like.
In this section, we give some details about how the modelling works under the hood for each of these 3 cases.
We use the terms “probability” and “likelihood” interchangeably throughout this section and denote them both by “p” in the math.
Modelling simple sessions:
Let us define an example session to be session = [A, B, C]
Then by applying the chain rule and Markov assumption, we can model the likelihood of the session as follows:
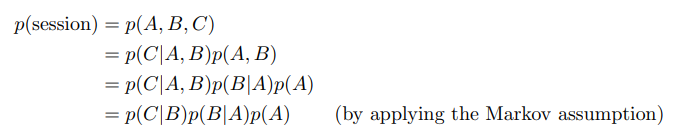
The Markov property is when the conditional probability of the next state depends only on the current state and on none of the states prior to the current state.
We define a transition probability as the probability of going from the previous event state to the current event state and we denote it as follows:

A subtlety to note is that we prepend and append start and end tokens respectively to each session by default. So, the start event A would be conditioned on the start token and we would have an additional transition probability in the likelihood calculation of the session terminating given the last event, C in the session.
So, to calculate the likelihood of a session, we would simply need to multiply a sequence of transition probabilities together. These transition probabilities can be estimated from the sessionized data as follows:

The likelihood calculations for longer sessions (more events) involve multiplying more transition probabilities together. Because the transition probabilities are between 0 and 1, this likelihood calculation will converge to zero as the session length gets longer. This could result in sessions being flagged as rare/anomalous simply because they are longer in length. Therefore, to circumvent this, we use a sliding window to compute a likelihood score per session.
Let us consider the following session = [A, B, C, D]
Let us also fix the sliding window length to be 3. Then we would compute the likelihoods of the following windows:
- [A, B, C]
- [B, C, D]
- [C, D, ##END##]
And then take the likelihood of the lowest scoring window as the score for the full session.
Notice that we are still using a start token in addition to the end token shown. The end token means we include an additional probability for the session terminating after the final event D. Whereas the start token appears implicitly when we condition the first event A on the start token.
It is important to note that if you choose a window length of k, then only sessions which have at least k-1 events will have a likelihood score computed. The -1 is because sessions of length k-1 get treated as length k during the scoring. This is due to the end token being appended before the likelihood score is computed.
This sliding window approach means we can more fairly compare the scores between sessions of different lengths. Additionally, if a long session contains mostly benign activity except for a small window in the middle with unusual malicious activity, then this sliding window method should hopefully be able to capture it in the score.
Okay, so this seems good. But what if most of our sessions are just the same few sequences of events repeated? How can we differentiate between these sessions?
Modelling sessions with parameters
Some of the Powershell cmdlets appear extremely often in our data in a seemingly scheduled automated way. The “Set-Mailbox” cmdlet is an example of this. This means we can end up with many identical sessions containing the exact same sequence of cmdlets. It can be seen here that the “Set-Mailbox” cmdlet can accept many different parameters. If we include the parameters in the modelling of the sessions, then it can help us to differentiate between the automated benign usage of a cmdlet and a more unusual usage.
Let us define an event as:

Where ci is the cmdlet used in the ith event of the session and each pj is either 1 if that parameter has been set for ci or 0 if not.
For example, suppose ci is the “Set-Mailbox” cmdlet and suppose that across all our sessions data, the only distinct parameters we have seen used for this cmdlet are [“Identity”, “DisplayName”, “ForwardingSmtpAddress”]. Suppose then that “Identity” and “ForwardingSmtpAddress” were set for this event, but the “DisplayName” parameter was not specified, then the parameter vector for this event would be [1, 0, 1].
Let us denote the parameter vector by {pk}i as a shorthand.
We now model the probability of the current event conditional on the previous event as follows:
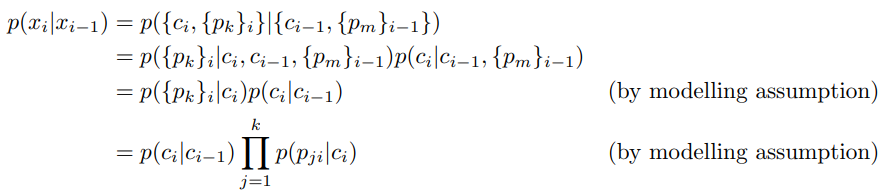
We made the following modelling assumptions:
- The parameters {pk}i used for the current event depend only on the current cmdlet ci and not on the previous event xi-1
- The current cmdlet ci depends only on the previous cmdlet ci-1 and not on the previous parameters {pm}i-1
- The presence of each parameter pji are modelled as independent Bernoulli random variables, conditional on the current cmdlet ci
We can estimate the probability of a parameter being used for a given cmdlet from our sessionized data as follows:

So now we can calculate the probabilities of the parameters conditional on the cmdlets and also the transition probabilities as before. The likelihood calculation for a session now involves multiplying a sequence of probabilities p(xi|xi-1) together where each p(xi|xi-1) can be decomposed as shown above. We also use the sliding window approach as before so we can more fairly compare the likelihoods between sessions of different lengths.
A subtlety to note is that in our implementation of this in msticpy, we take the geometric mean of this product:
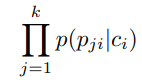
This means we raise the product of probabilities to the power of 1/k. The reason for this is because the cmdlets can have a vastly different number of parameters set on average. By taking the geometric mean, we can have a fairer comparison of how rare sets of parameters are across the different cmdlets.
Now we move on to describe how we implement the model for the case where the values of the parameters are modelled alongside the parameter names.
Modelling sessions with parameters and values
Some of the PowerShell cmdlets can accept parameters which have higher security stakes. For example, the Add-MailboxPermission cmdlet has an “AccessRights” parameter which can accept values such as “ReadPermission” and “FullAccess”. Because the “FullAccess” value could be used by an attacker for privilege escalation, it could be worth including the values of the parameters in the modelling of the sessions.
However not all the values are going to be useful in the modelling since parameters such as “Identity” can take arbitrary strings as their values. We therefore use some rough heuristics to determine which parameters take values which are categorical (e.g. high, medium, low) as opposed to arbitrary strings. We only include the values in the modelling for parameters which have been deemed suitable by the heuristics. However, there is the option to override the heuristics in the Model class directly.
This time, we denote an event as follows:

Where ci is the cmdlet used in the ith event of the session, each pk is is either 1 if that parameter has been set for ci or 0 if not and each vk is the value set for the parameter pk (if the parameter was set).
We now model the probability of the current event conditional on the previous event as follows:
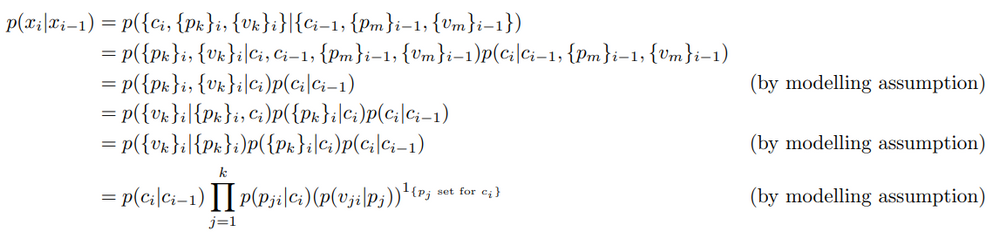
In addition to the modelling assumptions from the previous section, we assume the following:
- The values {vk}i depend only on the parameters {pk}i and not on the cmdlet ci
- The values vji are modelled as independent categorical random variables conditional on the parameter pj
- The probability of the value vji conditioned on parameter pj is only included if the parameter was set for cmdlet ci
We can estimate the probability of a value conditional on the parameter as follows:

A subtlety to note is that in our implementation of this in msticpy, we take the geometric mean of this product:
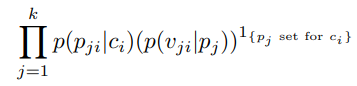
However, whilst in the previous section we raised the product of probabilities to the power of 1/k, this time we raise it to the power of:
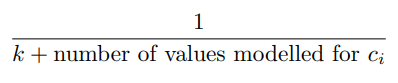
The reason for the modified exponent is so we can more fairly compare how rare a set of parameters + values are between the cases where some values are categorical vs arbitrary strings.
Some further notes
There are a few more details about our implementation which are worth mentioning. parameters and values during the model training.
where we add 1 to each of the counts that we observed in the data. For example, if this transition sequence of cmdlets “Set-Mailbox” –> “Set-User” appeared in our sessionized data 1000 times, we would use 1001 as the count instead. The reason for this is so when we estimate the probabilities from the counts, we shift some of the probability mass from the very probable cmdlets/parameters/values to the unseen and very unlikely cmdlets/parameters/values.
By including the ‘##UNK##’ token, we can handle cmdlets/parameters/values that we have not previously seen and are therefore not included in the trained model. Suppose that after the model training, we have some new sessions with cmdlets, parameters or values that were not included in the data from which we trained our model. We would like to use the trained model to compute the likelihood scores for these new sessions as well. Suppose a new session contains an unseen transition sequence such as “Set-User” –> “Some-UnseenCmdlet”. Then during the likelihood score calculation, when trying to access the probability for the unseen transition, the probability for this transition will be used instead: “Set-User” –> “##UNK##”. Now although we would not have encountered “Set-User” –> “##UNK##” during the model training, because of the Laplace smoothing, this transition would have a count of 1 instead of 0, and would therefore have a small non-zero probability.
This means we can train a model on some historical data, and then use the trained model to score new without the model raising exceptions for new previously unseen cmdlets/parameters/values.
The Laplace smoothing and usage of the ‘##UNK##’ token is applied by default and is currently nonoptional. We hope to make it optional via a configurable argument in the Model class in a future release of msticpy.
Summary
By combining various data science techniques, we sessionized, modelled and visualised our Office 365 Exchange so that we could identify anomalous user sessions. We used msticpy and Jupyter notebooks to perform this analysis, leveraging the high-level functions inside the anomalous_sequence subpackage. We then did a deep dive into the underlying implementation for each of the model types. The methods outlined in this blog can be applied to other security log types in a similar way to aid with threat hunting.
by Scott Muniz | Jun 29, 2020 | Uncategorized
This article is contributed. See the original author and article here.
This blog post was written by MVP Keith Whatling as part of the Humans of IT Guest Blogger series. Keith describes how giving back will help put a smile on your face.
If you’re already living the dream… how do we help people in tech poverty, the tech have nots?
When anyone thinks of donations or charity, our perceptions of what charity is kicks in. Wherever we look in the western world, people are after our help. The faces of starving children in far-flung war-torn countries stare down at us from the advertising boards on public transport, sandwiched in between the advert for the latest miracle hair remedy or a start-up offering to make our money go further. We walk through our towns to be confronted by gangs of clipboard wielding youngsters trying to grab our bank details for a poultry direct debit for one charity or another. They will even send you photos of the Goat you sponsored as a way of thinking that your £3.00 a month actually made a difference. No matter how hard a person I try to be, there is always something in me that feels bad that I did not sign up for every last clipboard offering! This is one of my constant first world problems.
This is what I know
I do, however, have another first world problem and that is learning technology. I’ve had a computer in some form since the tender age of 7, which seems like such a long time ago. Back then it was easy to master the beige box, tinted by the glow of the green screen, all sat on a special table in the spare room, soon to be called the “Computer Room”! That was then. Now, I work in the cloud, on someone else’s computer, and what was once for making 4 voice polyphonic music, 8-bit art or painstakingly typing in a game from the bi-monthly editions of BBC Micro magazines, it has now become my career. My weekends are spent in the garden, trying to distance myself from technology. That said, something was missing in my life. That something was giving back.
I’ve been so lucky to have been able to dip in and out of technology over the years, I’ve sold door to door, I’ve driven a bus, cleaned gardens and delivered appliances. Yet in other jobs, I have done graphic design, data analysis, run machine learning projects and lately I’ve been on the Low Code – No Code bandwagon with Microsoft Power Apps and the Power Platform and yet not a developer. I first learnt about charities needing tech way back in the late ’90s when charities needed a web presence. They wanted a look and feel on par with their printed material. Having a website made them feel included in the then .com boom and helped them expand their reach.
Where is my app?
Fast forward 20 years and the need for a website is now replaced with “I need an app”. In my mind, I see CEOs at some posh restaurant all sitting down for dinner and comparing apps – well, at least half the table is, while the other half have not been able to justify the development cost of their app. Yet now even as I speak there are businesses with thousands of PowerApps! This democratization of tech has given us an incredible opportunity and a considerable problem of the Tech Haves and Have-Nots at the same time.
In 2019 Satya Nadella stood at the Microsoft Build conference and said “500 million apps will be built between now and 2023”, which is no small number. Yet it was only 8 months prior at Microsoft Ignite that Satya’s keynote included “AI for Good”. I was blown away, yet at the same time there was one image from the presentation that stuck with me: the person handing out aid as part of this AI revolution stood there in a crowd of people with a clipboard and paper. I was confused. How can we get it so right and yet so wrong? How can we be providing modern AI tech and still be dependent on old-school paper processes? It just did not make sense to me.
Hacking For Good
I did not realize that a blog on my confusion and subsequent changes would lead to getting involved in the Hack4Good movement. In a nutshell, Hack4Good are hackathons with a charitable theme and using your tech superpowers to do some good. These are just as much learning days for all involved, from novice to expert, as they are a way to connect charities with people who want to help. The first one I took part in was in 2019 in London. It was epic but there was a problem of continuity. Just like any good superhero, it’s only when our powers combine like a giant Gundam that we start to smash the crux of the problem. You can read more about Hack4Good examples at https://techcommunity.microsoft.com/t5/humans-of-it-blog/guest-blog-hacking-the-power-platform-for-good/ba-p/1416160.
My biggest problem
I’m dyslexic. I hate reading instructions because I always miss something. I learn by doing and watching videos. But the “doing” is always a problem. I need a use case to practice on. I’ve come to learn over the years that a use case that I come up with is biased towards what I can do, rather than what I cannot! I need a real-world use case, with a client who is going to use the “And” operator to ask me to do a bit more over and over.
Welcome COVID-19
Our current global predicament has spawned thousands of Power Apps worldwide! Businesses are using apps to communicate with their staff, manage knowledge, book desks for a socially distanced office and track contacts. Hospitals are using them to capture stats and, in some cases, have built bespoke extensions to Microsoft’s amazing Emergency Response tool kit. In fact, a friend of mine is calling Coronavirus his Chief Digital Transformation Officer as they have managed to get done in 2 months what they could never have done in years. To quote John Cleese in The Day the Earth Stood Still, “It’s only at the brink that people find the will to change, only at the precipice to we evolve”. I think about the quote a lot these days.
A puppy is not just for Christmas.
Every technologist knows that EVERY TIME they return to their family home or see that Auntie, there are going to be those standard tech support questions, like “Can you fix Mum’s phone, fix the Wi-Fi, my computer is slow etc.” – you need to be there long term.
The issues with helping for just one day is just like going to see a relative. We help with a small part of the problem. It’s a bit of a “teach a man to fish” issue. What might be needed is true adoption. I don’t mean the tech adoption that we see where the IT department roll out Microsoft 365 to staff who will just continue to save their Excel workbooks to their USB drive. No, I mean if we the makers and masters of tech were to adopt a charity, a local business or just to take a “Tech-have-not” and spend time with them, accept them, have the patience to take them on a learning journey, then we can really help drive sustainable change and ensure they can continue in the new normal.
Up the wizard’s sleeve
A thousand years ago people who knew the secrets of something were wizards, wise women or witchdoctors – we have some fanciful notion of them travelling from place to place doing good for free. Is this us giving away our skills for free? Nothing could be further from the truth!
No amount of Microsoft certifications will be as impressive to a prospective employer or client than an actual portfolio of work. If you have worked in one sector then your portfolio might be limited. Adoption of a business or a charity, partnering with your local nonprofit a few hours a month to build an app or sit on a call to guide and advice others will give you another page in your portfolio that you can be proud of. It will make the world a better place and enable you to peacefully walk past the sea of clipboards in the Highstreet knowing that you are giving way more than a faceless 3 quid.
So what am I asking for? Reach out and teach one person tech, take a Tech-Nobody and teach them without limits.
Adopt a local business. It could be the local shop, a tree surgeon, a small supplier. Take them on the journey and make it a project. A lot of small businesses are hurting right now, and need tech to help to transform their businesses in the new normal.
Group together – become the unstoppable giant team robot and for a collective of awesome that offers to help others!
No matter what you choose to do, what ultimately matters is that you do something. You’ll feel better for it! And hell you might even get a better job at the end of it!
#HumansofIT
#TechforGood
Recent Comments