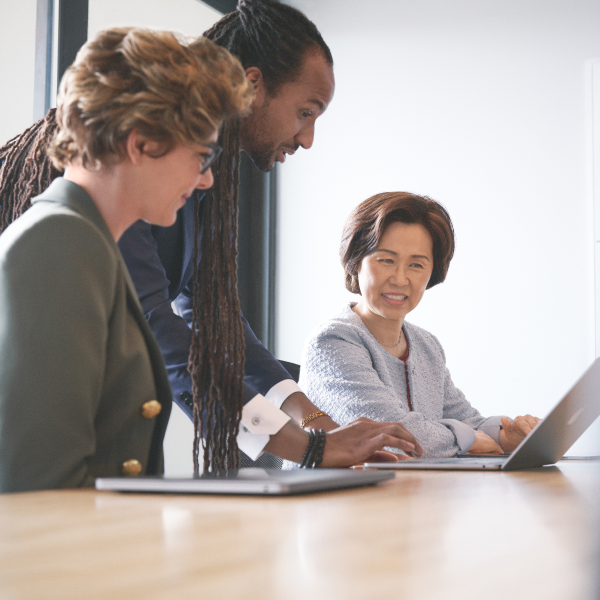
Elevating experiences with AI, from productivity to personalization
This article is contributed. See the original author and article here.
In a previous blog, we discussed how modern, AI-enabled customer relationship management (CRM) platforms and enterprise resource planning (ERP) systems help drive new, more effective ways of working for employees and more satisfying outcomes for the customers they serve in three key ways: by streamlining operations, by empowering more informed and insightful decisions, and by elevating customer and employee experiences.
In this blog post, we’re going to focus on the third item: elevating customer and employee experiences by showing how AI-enabled CRM solutions help increase productivity and provide unprecedented levels of personalized service across three key business functions: marketing, sales, and customer service. We’ll also provide insights and best practices for how to help employees get the most from AI, including how they can be empowered to create personalized experiences for their customers.
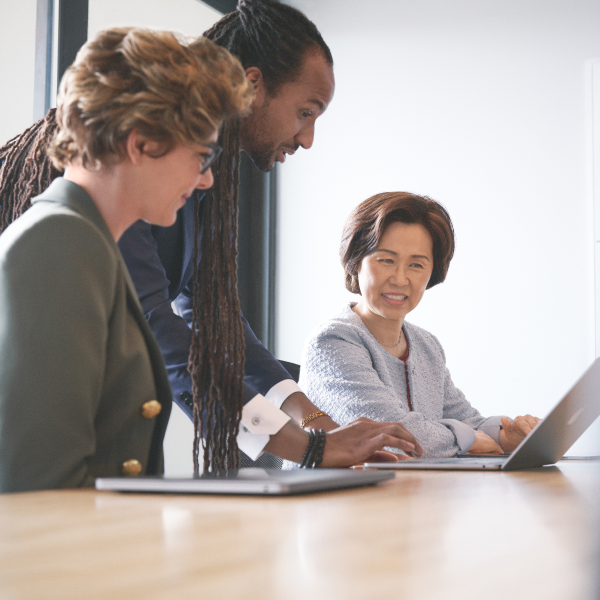
Revolutionizing marketing: How AI-enabled CRM software drives personalized experiences and enhance customer engagement
Marketing is one area where generative AI is already in active use. According to a Forrester survey of CMOs, more than half (56%) of B2C marketing or advertising decision makers have been using generative AI in three key ways:
- To help employees minimize tedious tasks, allowing for more time to focus on strategically important work.
- To summarize insights and enable swift action without the need to dig through data manually.
- To boost the scale of creative output by generating starter ideas along with visuals and copy.1
AI is particularly capable of delivering personalized experiences in marketing, where AI-enabled CRM platforms can marry customer data to messaging to create memorable moments and impact sales. One example of a company using AI-enabled CRM solutions to generate marketing content is North Carolina-based sports club NC Fusion, which used Microsoft Copilot in Dynamics 365 Customer Insights to help its marketers create personalized messaging tailored to its audience segments, increasing the reach of the brand. Using AI-enabled content ideas, descriptions, and summaries has provided significant time savings, and personalizing campaigns has been more effective with Copilot.2
“For families, we are able to tailor the message they receive. This means a family will only receive messages that apply to their situation, and not a multitude of emails that have no application to their family situation. With AI-assisted content production, our customer engagement has increased from 10% to 30%.”
Chris Barnhart, Head of IT and Data Systems at NC Fusion
Empowering sales teams: How AI-driven personalization can transform customer interactions and boost revenue
Another area where personalization can impact an organization’s bottom line is sales, where making authentic connections with customers at the right time is paramount. Few organizations know this better than superyacht brokerage Northrop & Johnson, which has used AI to deliver highly personal sales experiences tailored to the wants and needs of its high-value clients.
“In this market, we have high-wealth customers who are considering very high-value purchases, and we can’t afford any interactions that leave them feeling anything less than special.”
Keith Perfect, Director of Technoloy & Intelligence at Northrop & Johnson
Microsoft Dynamics 365 Sales, Microsoft Dynamics 365 Customer Insights, and Copilot provide Northrop & Johnson sales teams with comprehensive and timely data for each client, which helps them deliver personalized conversations at precisely the right time to engage.
“Clients at this level want to know they are taken care of. And when you must make an impact in minutes, which is all you have with these busy clients, you need to be very attuned to them. Otherwise, it could cost you the sale. So, having a solution at your fingertips that connects the entire journey is huge for our sales team.”
Daniel Ziriakus, President & Chief Operating Officer at Northrop & Johnson
Sales teams using AI-enabled CRM software also realize significant time savings as salespeople assign more tedious tasks to their AI assistants. In fact, according to new Microsoft research, 79% of Microsoft Copilot for Sales users say it reduces the amount of administrative work they have to do, 67% say it helps them spend more time with customers, and 64% say it allows them to better personalize customer engagements.3
Transforming customer support: How AI-driven assistants enhance productivity, satisfaction, and retention
Customer service is still another area where AI-enabled CRM platforms can make an immediate impact. According to a November 2023 study from the National Bureau of Economic Research (NBER), customer service agents using a generative AI-based conversational assistant were able to increase productivity—specifically measured by the number of issues resolved per hour—by an average of 14%. The effect was even more pronounced with novice and low-skill workers, who experienced productivity increases of 34%. Researchers also found that AI assistance improves customer sentiment and increases employee retention.4
One company using an AI-enabled CRM solution in customer service is Microsoft. We operate one of the largest customer support teams in the world and process more than 145 million contacts per year. We use Microsoft Dynamics 365 Customer Service to help utilize the full expertise of the engineers on staff and provide better resolution of customer issues across the board.5
“The challenge for every support engineer is to connect with the human being on the other end of the call who has a problem that needs solving. You want to connect with them, but you also need to be able to pull in a great deal of technical information. Copilot provides us the support to offer the customer understanding while also sorting out their technical problems.”
Ric Todd, Director of Support Strategy at Microsoft
Download The AI Advantage: Driving sales performance with next-generation tools.
Bringing AI to your organization
For leaders looking to roll out AI solutions in their organizations, we have some encouraging news: people new to AI begin recognizing its value quickly. Recent Microsoft research shows it takes a time savings of just 11 minutes per day for most people to see its usefulness (a key factor in getting new work habits to stick). 6
Encouragingly, most respondents report having saved more than 11 minutes. The most efficient among them are saving up to 30 minutes per day—the equivalent of 10 hours per month—and the average person is saving 14 minutes per day for a time savings of almost five hours per month.
What’s more, the breakthrough moment by which respondents report seeing improvements in productivity (75%), work enjoyment (57%), work-life balance (34%), and the ability to attend fewer meetings (37%) happens within one business quarter—11 weeks.7
While personal productivity gains from Copilot are real and significant, building an AI-powered organization requires committing to working in a new way. Some best practices to consider include:
- Encourage daily use. Realizing productivity gains from AI will take intentional everyday practice. Those who start building the habit early will pull ahead. And don’t forget—11 weeks is all it takes for people to recognize the effect.
- Help people manage their new assistants. Employees taught to treat their generative AI tools as assistants, not search engines, will get the most value. Teach team members to manage their new assistant and to recognize when to delegate a task to AI and when to apply their human intelligence, judgment, and skill.
- Find good use of reclaimed time. Help your team take advantage of time savings to focus on the higher-order and creative tasks only people can do. Salespeople can devote more time to building relationships with customers and closing deals. Marketers can carve out time to dream up new solutions. Customer service teams can focus on solving problems, and managers across the organization can spend more time coaching and caring for their teams.
Taking the next step forward
Take the next step in your AI adoption journey by learning more about Copilot and other AI-powered capabilities in Microsoft Dynamics 365. Discover how to keep your organization on the cutting-edge by realizing that a new paradigm of customer engagement through AI-enabled personalization empowers both customers and employees.
Join us at a Microsoft Dynamics 365 Virtual Training Day to gain the skills needed to help your organization sell, service, and deliver on the customer expectations of tomorrow. Register for free, in-depth training events, where you’ll uncover new efficiencies of scale, discover smarter connections, and utilize built-in intelligence for deeper insights into your business. Register now!
Microsoft Dynamics 365 Virtual Training Day
Join us at a Microsoft Dynamics 365 Virtual Training Day to gain the skills needed to help your organization sell, service, and deliver on the customer expectations of tomorrow. Register for free, in-depth training events, where you’ll uncover new efficiencies of scale, discover smarter connections, and utilize built-in intelligence for deeper insights into your business.
Sources
- Leap Now, Not Later, into a Responsible Generative AI Strategy for Marketing (July 5th, 2023)
- Microsoft Customer Story-NC Fusion expands youth marketing outreach, taught kids leadership and life skills with Dynamics 365 Customer Insights
- What Can Copilot’s Earliest Users Teach Us About Generative AI at Work? (microsoft.com)
- Working Paper 31161; Generative AI at Work; NBER
- Microsoft Customer Story-Microsoft empowers support engineers to shine brighter with Dynamics 365 Customer Service
- AI Data Drop: The 11-by-11 Tipping Point
- AI Data Drop: The 11-by-11 Tipping Point
The post Elevating experiences with AI, from productivity to personalization appeared first on Microsoft Dynamics 365 Blog.
Brought to you by Dr. Ware, Microsoft Office 365 Silver Partner, Charleston SC.
Recent Comments