by Contributed | Oct 6, 2020 | Azure, Technology, Uncategorized
This article is contributed. See the original author and article here.
Azure Sentinel, among the most advanced SIEM solutions, is deeply infused with Machine Learning (ML), providing unparalleled richness of built-in, advanced ML analytics, covering the prevalent threats and data types connected to the SIEM. Now, the same richness of capabilities is made available to the data scientists in organizations, extending the reach to unique customer threats, providing Azure Sentinel customers the ability to build their own ML models.
Built-in analysis using machine learning, like ‘Fusion’ ML detections and entity enrichment, is already available in Azure Sentinel, identifying advanced threats on well-known data feeds, while maintaining a low level of alert fatigue. See this blog to learn more about Fusion.
Many organizations need to extend the advanced analysis capability to the myriad of threats applicable to their organization or industry vertical. Azure Sentinel makes it easier for data scientists in these organizations to unlock these insights with a BYO-ML framework.

Azure Sentinel provides a threat detection framework for custom ML, including platform, tools, and templates, to accelerate development of models for custom business analysis, leveraging Microsoft’s shared algorithms and best practices.
Azure Sentinel integrates its bring-your-own-ML feature with familiar development environments, tools, and programming languages, like Azure Databricks, Spark, Jupyter Notebooks and Python, to reduce the learning curve and development time to the minimum. The framework comes with cloud scale data pipelines, seamlessly integrating the ML development and runtime environment with Azure Sentinel service and user experience, significantly reducing the time to value from custom ML analysis.
Using the BYO-ML framework, customers can:
- Train an ML algorithm shared by MS or community with your own data, to customize the ML model to your environment
- Modify an ML algorithm and/ or features to customize the model, adapting it to your organization
- Create new model from scratch, or import existing models, leveraging Azure Sentinel’s BYO ML framework and tools
- Operationalize the model in Databricks/ Spark environment, leveraging the framework to seamlessly integrate your ML environment with Azure Sentinel user experience and operational flow (detections, investigation, hunting and response)
- Share the ML algorithm for the community
This article provides an overview of BYO ML framework and recommended usage of its components. For detailed documentation please refer to Azure Sentinel feature documentation.
Azure Sentinel features of the BYO-ML framework
The BYO-ML framework includes the following features:
- Anomaly detection model template: an example of an end to end template for a generic algorithm for ‘access to a network share’ anomaly. The example is provided with pre-trained data on Windows file share access log, that a customer is encouraged to retrain on their custom data.
- Template for Model Training: an example of notebook to train the algorithm, build and save the model
- Template for Model Scoring: an example of notebook to run the model on schedule, scoring new data
- Data pipelines between Sentinel and ML environments: used for exporting Azure Sentinel data to the Spark execution environment for model training and scoring and importing the scored results back into Azure Sentinel tables
- Utilities for integration with Sentinel: support for enabling the integration between the environments
- Enrichments: making entity enrichment information like peer metadata and blast radius available to the custom ML models for refinement
The BYO ML package shared in Github, includes utilities and a model example showcasing the best Microsoft ML practices and research for security.
BYO-ML environment for Azure Sentinel
To build custom ML models on your data, you have 2 options.
For smaller amounts of data, like alerts and anomalies, you can use Azure ML to run models hosted in the Azure Sentinel Notebooks (new menu option currently in Preview).
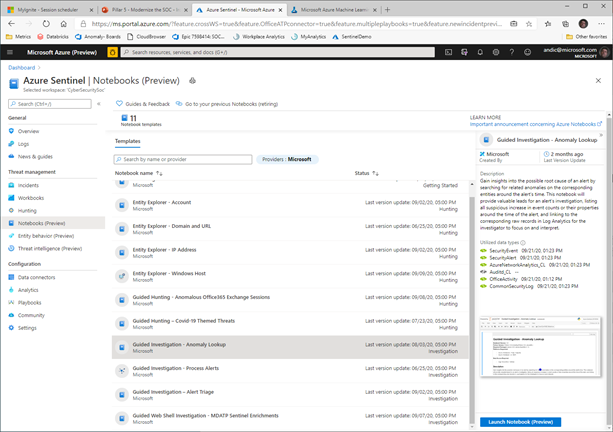
The Azure ML experience is launched from this Azure Sentinel experience, with the selected Notebook loaded in the ML environment, operating to the data in the Azure Sentinel tables.
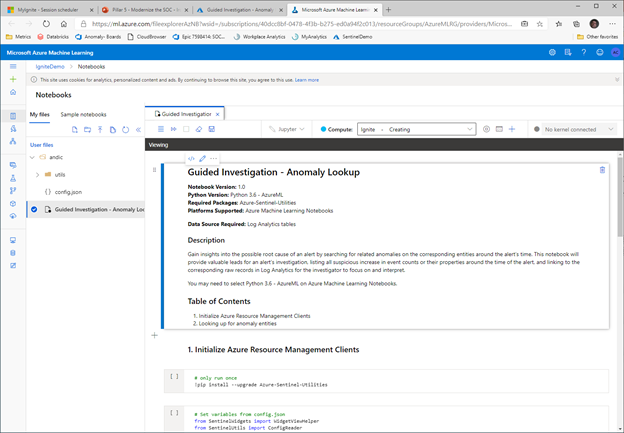
You can use this option on a small sample of large data as well, to ease the initial steps of development.
For development and operationalization of models built on larger data, like analyzing feeds of raw data, you will need to make this data accessible to the ML model in Azure Databricks.
Apache Spark™ provides a unified environment for building big data pipelines. Azure Databricks builds on this environment, providing a zero-management cloud platform, holistically addressing the platform needed for data analysts to develop their custom ML based security analysis.
You can either bring your raw data directly to the Azure Databricks ML environment, via EventHub or Azure Blobs or you can use the capabilities provided with Azure Sentinel, to export the data from Azure Sentinel Log Analytics tables. Regardless of the export methods used for raw data, you can use the libraries provided by BYO-ML framework to import the scoring of the ML model back into Sentinel Log Analytics tables for further processing and creating incidents.
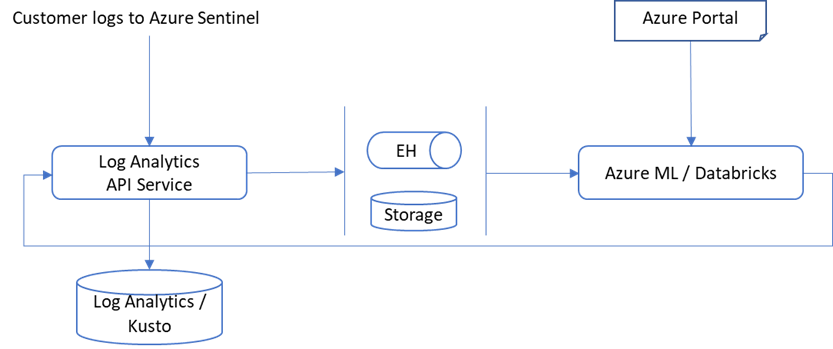
On Azure Sentinel roadmap, we plan to support Azure Synapse in addition to Azure Databricks as the BYO-ML development environment.
You can either set up a new Azure Databricks environment or use one already set up for other use. To set up a new Databricks environment, please reference to the quickstarts document (note that MMLSpark used by our algorithm requires Spark 2.4.5).
Once the environment is set, export your data from Azure Sentinel into the BYO-ML environment, using the Azure Command Line Interface (CLI). See Azure Sentinel BYO-ML feature documentation for details.
BYO-ML Example Walkthrough: Anomalous File Share Access Detection
The BYO ML package includes an example of an ML model, leveraging the framework to deliver a customized ML detection.
The example is an ML algorithm template for “anomalous resource access” detection. It is based on collaborative filtering algorithm, trained and tested on Windows file share access logs, containing security events of users and resources accessed by them (Event ID 5140).
The example includes a notebook used to train the model (AnomalousRATraining.ipynb) on your specific organization data.
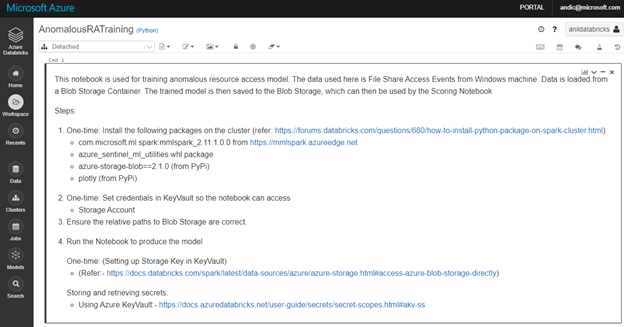
An additional notebook, AnomalousRAScoring.ipynb, allows you to adjust the model features to the anomalous behavior and accepted level of noise in your organization. You can use it to evaluate the scoring results, adjusting model thresholds, filtering out known benign users and ranking the anomalous ones.
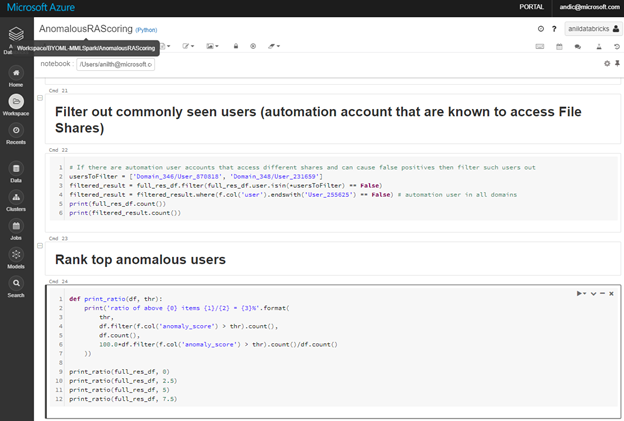
Optionally use visualization capabilities in the Notebook, to check the results of the iterations and adjust to an acceptable noise level.
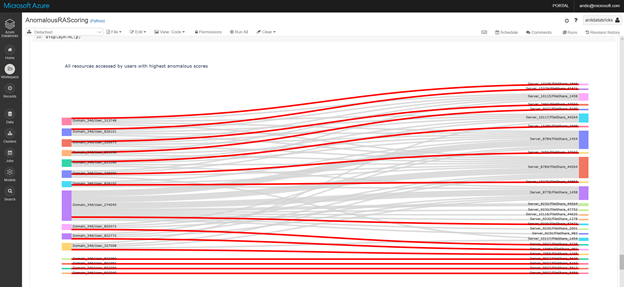
Once you are satisfied with the scoring results, use the same Notebook to write the results back to the Log Analytics workspace associated with your Azure Sentinel instance.
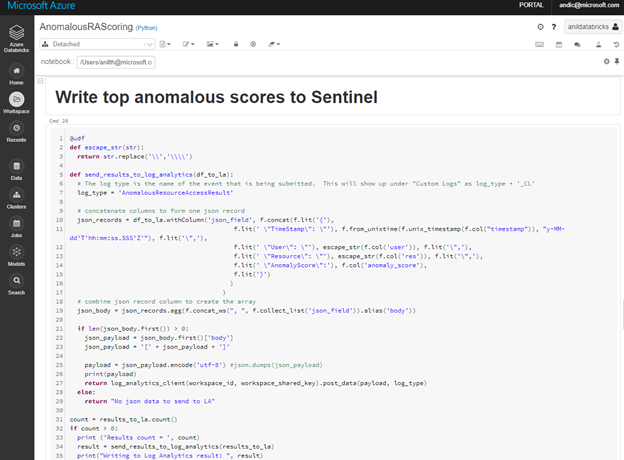
With the scored data in the Azure Sentinel tables, you can use the standard analytics development experience to create your custom rule(s), as you would do for the rest of your custom KQL queries.
BYO-ML is currently in Public Preview. If you have any questions or feedbacks, please reach out to askepd@microsoft.com.
by Contributed | Oct 6, 2020 | Azure, Technology, Uncategorized
This article is contributed. See the original author and article here.
Microsoft Azure SQL Edge, now generally available, and DH2i DxOdyssey for IoT are combining native on-device capabilities with dynamic tunneling technology for an optimal IoT edge security solution.
It’s no news that IoT devices are generating more and more of data, but did you know that ? *
With so much data being created at the edge, security is one of the top concerns for IoT adopters. In a recent study, 97% of IoT adopters have security concerns when implementing IoT, and the top concern was ensuring data privacy.** Data needs to be protected both at the edge and as it moves outside the device boundaries to edge networks and other external networks and devices.
Azure SQL Edge is a small footprint data engine optimized for IoT workloads.
Built on the same code base as Microsoft SQL Server and Azure SQL, Azure SQL Edge provides the same industry leading security, familiar developer experience, and tooling that many teams already know and trust – now extended to IoT deployments for real-time intelligence.
Thanks to this shared codebase, application developers who have been building solutions for SQL Server and Azure SQL can extend their skills to deploy the same code to build IoT solutions at the edge that work with or without network connectivity.
Azure SQL Edge answers the need for an IoT-specific data platform by:
- Providing the flexibility to develop solutions that work with or without network connectivity and help enable secure data movement of the local edge data to on-premises datacenters or to the cloud.
- Offering support with standard tooling, programming languages, and a query language (T-SQL) that are already familiar to developers and compatible with existing code.
- Enabling artificial intelligence (AI) and analytics at the edge.
- Including native support for ingesting and streaming time-series data.
Unparalleled database security of the Microsoft SQL data engine.
The same security features of SQL Server Enterprise available in Azure SQL Edge ensure your data is secure on the device, customer data will be kept safe, and regulatory compliance will be met.
Here are the built-in security features that you won’t have to worry about in your solution thanks to Azure SQL Edge:
- Role-based access control (RBAC) and attribute-based access control (ABAC) to manage access to specific resources based on the user’s group (or role) and/or based on the attributes of the user, target data or resource
- Data protection with the ability to encrypt sensitive data and execute rich computations on encrypted data with Transparent Data Encryption (TDE) and Always Encrypted capabilities
- Sensitive PII/GDPR data discovery using SQL Data Discovery and Classification tool in SSMS
- Data classification to help organizations comply with security regulations by allowing data to be categorized by sensitivity and business impact
Azure SQL Edge extends the security of Azure and SQL Server to the Edge, protecting your data within the database and the Azure IoT Hub infrastructure.
Enhanced secure data movement with DxOdyssey
Beyond the security of the Azure SQL Edge and Azure IoT Hub network, Microsoft has partnered with DH2i to support enhanced security of data movement with its Software Defined Perimeter (SPD) software, DxOdyssey for IoT. DH2i is a software vendor with over 10 years of experience helping customers around the world enhance their data security and SQL Server capabilities with their innovative software offerings. Where common approaches to networking such as VPNs, open ports and SD-WAN are insufficient and lack the security capabilities required to support IoT deployments, DH2i’s DxOdyssey (DxO) for IoT extends SDP software capabilities to edge devices, allowing seamless bi-directional access from edge devices to the datacenter and cloud. As data moves outside of edge device perimeters and between networks, DxO for IoT ensures the data remains secure.

DxO for IoT is purpose-built for IoT use cases where bi-directional communication is needed between edge devices such as sensors, edge gateways or edge servers. Its technology:
- provides discreet, private and secure network communication over untrusted networks, such as the public internet.
- eliminates the lateral network attack surface and is more secure and performant than VPNs, open ports or SD-WAN.
- creates a Zero Trust network architecture for IoT devices and applications while consistently proving less expensive, easier and more effective than those legacy alternatives.
This lightweight software runs on any Linux or Windows host and can be installed on any IoT device or container on x64 and ARM 64 architecture.
When used in tandem with Azure SQL Edge, organizations can ensure their data is secure on the edge device and when passing outside of edge networks and IoT Hub boundaries.
Join us on October 21 to deep dive into edge security and learn about Azure SQL Edge and DxOdyssey for IoT including a demo of how to securely connect a remote user to an edge device, Register Now
* “Edge Computing Solutions for Industrial IoT”, July 2018, Gartner, “Top Strategic IoT Trends and Technologies Through 2023” September 2018, Gartner
** “IoT Signals” September 2020, Microsoft.
by Contributed | Oct 6, 2020 | Azure, Technology, Uncategorized
This article is contributed. See the original author and article here.
Initial Update: Tuesday, 06 October 2020 19:55 UTC
We are aware of issues within Application Insights and are actively investigating. Some customers in East US may experience intermittent data gap and incorrect metric alert activation starting 18:45 UTC.
- Work Around: NA
- Next Update: Before 10/07 00:00 UTC
We are working hard to resolve this issue and apologize for any inconvenience.
-Subhash
by Contributed | Oct 6, 2020 | Azure, Technology, Uncategorized
This article is contributed. See the original author and article here.
To provide customers with an easier network configuration, all newly created virtual clusters will be enabled for access over global virtual network peering connections, now in general availability. This enables customers to pair managed instances in failover group configuration, in an easy and performant way, by simply connecting virtual networks in different regions. By utilizing global virtual network peering for your managed instances, you will save time through easy network configuration and offload your gateways from database replication traffic. Review this new feature and more in this episode with Srdan Bozovic.
Watch on Data Exposed
Resources:
Virtual network peering
Auto-failover groups
View/share our latest episodes on Channel 9 and YouTube!
by Contributed | Oct 6, 2020 | Azure, Technology, Uncategorized
This article is contributed. See the original author and article here.
Although the capability to query the Secure Score using API was already available and we already published some automations to leverage this capability, now you can also query your Secure Score using Azure Resource Graph (ARG). In addition, you will be able to calculate the score for the security controls and accurately calculate the aggregated score across multiple subscriptions. The following tables were added to allow you to query not only the secure score, but also the security controls:
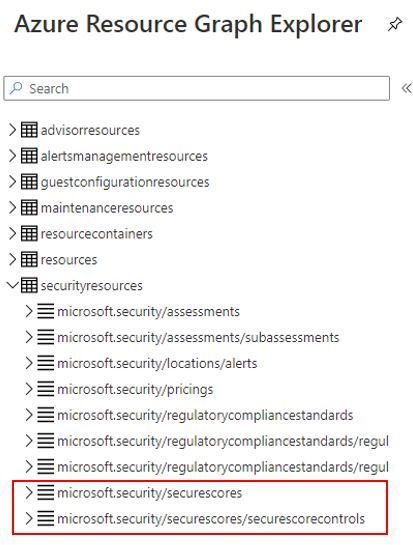
Here an example of how to query your current secure score (percentage):
SecurityResources
| where type == ‘microsoft.security/securescores’
| extend current = properties.score.current, max = todouble(properties.score.max)
| project subscriptionId, current, max, percentage = ((current / max)*100)
This query will return an output that looks like this one:
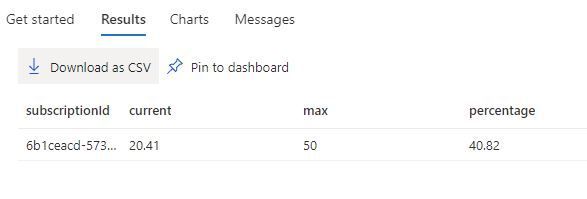
This corresponds to what you see in the UI for your subscription. In this case, ASC dashboard show the following Secure Score:

This other example below will list all security controls, the amount of unhealthy resources, their current score and their max score:
SecurityResources
| where type == ‘microsoft.security/securescores/securescorecontrols’
| extend SecureControl = properties.displayName, unhealthy = properties.unhealthyResourceCount, currentscore = properties.score.current, maxscore = properties.score.max
| project SecureControl , unhealthy, currentscore, maxscore
The output should look like the example below:
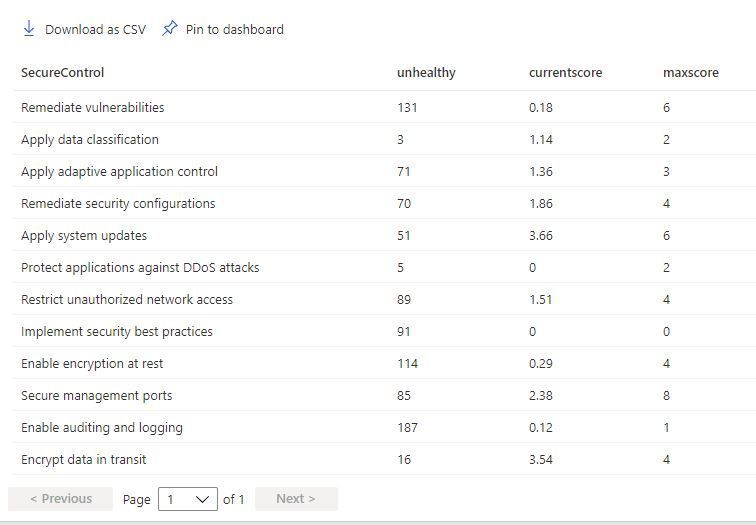
Calculating the Secure Score
For a single subscription the type of resource we are calling is the microsoft.security/securescores where we get the maximum score (∑ maximum scores of each security control) and the current score (∑ current scores of each security control). To obtain the secure score of a single subscription, the following formula is used:

To obtain the aggregated secure score for multiple subscriptions you should use the formula below:

As you can see in the formula, the aggregated score for multiple subscriptions is not the average, it uses the weight field that is provided in the query (ARG or API). The weight takes in account the size of the subscription (# of resources ) and hence allows to calculate a weighted score which is more accurate than a regular average between different subscriptions with different sizes. For more information refer to the secure score documentation page.
To see how this looks like, let’s use the query below as an example:
securityresources
| where type == “microsoft.security/securescores”
| extend subscriptionScore = todouble(properties.score.current)/todouble(properties.score.max)
| extend subScoreXsubWeight = todouble(subscriptionScore)*todouble(properties.weight)
| summarize upperValue = sum(subScoreXsubWeight), underValue = sum(todouble(properties.weight))
| extend overallScore = round(100*((upperValue)/(underValue)))
| project overallScore
The subscriptionScore is obtained dividing its current score by its maximum score. Then, the value subScoreXsubWeight holds the subscription secure score times the subscription weight. Finally, the overall score is the sum of subScoreXsubWeight divided by the sum of the subscriptions’ weights. The result is shown below:
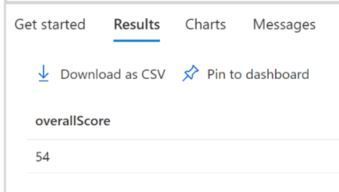
One of the advantages of querying the secure score via ARG is that you can quickly use these queries to create custom dashboard with Azure Workbooks. If you are querying the secure score using API, you can send a GET Request as shown below:
GET https://management.azure.com/subscriptions/20ff7fc3-e762-44dd-bd96-b71116dcdc23/providers/Microsoft.Security/secureScores/ascScore?api-version=2020-01-01-preview
In the JSON result you will see the weight field, which is used to calculate the secure score is also available as shown the sample below:
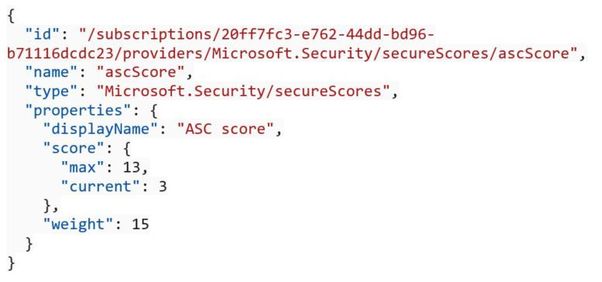
For more information about the Secure Score API, read the following articles:
Make sure to utilize this feature and provide your feedback in the comments below, or if you want to formally suggest improvements, provide your feedback at https://aka.ms/ascuservoice
Thanks to all Reviewers
Miri Landau, Senior PM – Azure Security Center Engineering Team
Meital Taran- Gutman, Principal PM – Azure Security Center Engineering Team
Fernanda Vela Huerta, PM – CxE Azure Security Center Team
by Contributed | Oct 6, 2020 | Azure, Technology, Uncategorized
This article is contributed. See the original author and article here.
Initial Update: Tuesday, 06 October 2020 06:03 UTC
We are aware of issues within Application Insights and are actively investigating. Some customers may experience failures while accessing metrics from the Azure portal.
-
Work Around: None
-
Next Update: Before 10/06 08:30 UTC
We are working hard to resolve this issue and apologize for any inconvenience.
-Vyom
Recent Comments