by Contributed | Oct 1, 2020 | Azure, Technology, Uncategorized
This article is contributed. See the original author and article here.
This year at NVIDIA GTC, we’re excited to expand on our announcement of new GPU VM sizes available to Azure customers that continue our promise of providing VMs for every workload, at any scale- from a few inferences, to training multi-billion parameter natural language models.
The first is a flagship scale-up and scale-out offering, built on the NVIDIA A100 Tensor Core GPU– the ND A100 v4 platform- for users requiring the next frontier of AI training capabilities, which we unveiled in August. Equally important, however, in rounding out the Azure AI GPU portfolio, is a new and efficient NVIDIA T4 Tensor Core GPU-equipped VM series. The NC T4 v3 VMs are a cost-effective yet powerful option for customers looking to deploy machine learning in real-time production contexts.
Azure is committed to offering our customers the performance, pricing, unique features and worldwide availability they need to be successful in the rapidly changing landscape of accelerated cloud infrastructure. Four other generations of NVIDIA GPUs are also available to our customers worldwide, with a dozen Virtual Machine size options, including the industry’s only public cloud VMs with NVIDIA Mellanox InfiniBand scale-out networking.
Flagship NVIDIA A100– powered Azure VMs for Demanding AI Training and Compute
During the AI and GPU Infrastructure at Every Scale in Azure session [A22245TW] at GTC, we’ll be disclosing more details around the NVIDIA A100-powered ND A100 v4 product for high-end GPU computing in the cloud. This brand new offering, built around eight NVIDIA A100 Tensor Core GPUs, is poised to fulfill the most demanding AI training requirements for customers with large models, and enable a new class of tightly coupled, distributed HPC workloads. With ND A100 v4, customers can harness the power of interconnected GPUs, each connected via PCIe Gen 4 with an independent NVIDIA Mellanox HDR 200 Gigabit InfiniBand link.
Scalable from eight GPUs and a single VM, to hundreds of VMs and thousands of A100 GPUs- a scale rivaling some of the world’s largest supercomputers- clustering is turnkey with out-of-box support for NVIDIA’s NCCL2 communication library. Distributed workloads benefit from topology-agnostic VM placement and a staggering 1.6 Terabits of interconnect bandwidth per virtual machine, configured automatically via standard Azure deployment constructs such as VM Scale Sets.
Designed in close partnership with NVIDIA, the ND A100 v4 supports the same industry standard Machine Learning frameworks, communication libraries, and containers commonly deployed on-premise as a standard Azure VM offering.
The ND v4 is in limited preview now, with an expanded public preview arriving late this year.
NVIDIA T4–Powered Azure VMs for Right-Sizing Cloud GPU Deployments
Also new to Azure are NVIDIA T4 Tensor Core GPUs, providing a hardware-accelerated VM for AI/ML workloads with a low overall price point.
The NC T4 v3 series provides a cost-effective, lightweight GPU option for customers performing real-time or small–batch inferencing, enabling them to right-size their GPU spending for each workload. Many customers don’t require the throughput afforded by larger GPU sizes, and desire a wider regional footprint. For these use cases, adopting the NC T4 v3 series with no additional workload-level changes is an easy cost optimization. For customers with larger inferencing needs, Azure continues to offer NVIDIA V100 VMs such as the original NC v3 series, in one, two and four GPU sizes.
The NC T4 v3 is an ideal platform for adding real-time AI-powered intelligence to services hosted in Azure. The new NC T4 3 VMs are currently available for preview in the West US 2 region, with 1 to 4 NVIDIA T4 Tensor Core GPUs per VM, and will soon expand in availability with over a dozen planned regions across North America, Europe and Asia.
To learn more about NCasT4_v3-series virtual machines, visit the NCasT4_v3-series documentation.
Azure Stack Hub and Azure Stack Edge is now available with GPUs
Azure Stack Hub is available with multiple GPU options. For example the NVIDIA V100 Tensor Core GPU, which enables customers to run compute intense machine learning workloads in disconnected or partially connected scenarios. The NVIDIA T4 Tensor Core GPU is available in both Azure Stack Hub and Azure Stack Edge and provides visualization, inferencing, and machine learning for less computationally intensive workloads. With NVIDIA Quadro Virtual Workstation running on the NVIDIA T4 instance of Azure Stack Hub, creative and technical professionals can access the next generation of computer graphics and RTX-enabled applications, enabling AI-enhanced graphics and photorealistic design.
Learn more about these recent announcements here: Azure Stack Hub and Azure Stack Edge.
Microsoft Sessions at NVIDIA GTC
Session Title
|
Location
|
Speakers
|
Session Type
|
Accelerate Your ML Life Cycle on Azure Machine Learning (A22247)
Azure Machine Learning helps scale out deep learning training and inferencing using distributed deep learning frameworks to parallelize computation with NVIDIA GPUs. See how you can scale your ML workflows in a repeatable manner and automate and drive cost efficiencies into the entire ML life cycle across model building, training, deployment, and management.
|
US
|
Keiji Kanazawa Principal Program Manager, Microsoft
|
Live
Tuesday, Oct 6, 08:00 – 08:50 PDT
|
Accelerating PyTorch Model Training and Inferencing with NVIDIA GPUs and ONNX Runtime [A22270]
Extend the Microsoft experience of training large natural language model with ONNX Runtime and Azure Machine Learning to your own scenarios.
|
US
|
Natalie Kershaw Senior Program Manager, Microsoft
|
On Demand
|
Visualization and AI inferencing using Azure Stack Hub and Azure Stack Edge [A22269]
Learn how you can use Azure Stack Hub or Edge to run machine learning models at the edge locations close to where the data is generated. We’ll demonstrate how to train the model in Azure or Azure Stack Hub to run the same application code across cloud GPU and edge devices and distribute these models to edge, using hosted services like Azure IoT Edge or as Kubernetes containers.
|
US
|
Vivek N Darera Principal Program Manager, Microsoft
|
On Demand
|
|
|
|
|
AI and GPU Infrastructure at Every Scale in Azure [A22245]
Join us for a brief walkthrough of Azure’s GPU-equipped infrastructure capabilities, from visualization to massively-parallel AI training supercomputers powered by the latest NVIDIA A100 GPUs and Mellanox HDR InfiniBand. From the cutting edge of AI research to production deployment of ML services worldwide — and any scenario in between — Azure has a VM for every workload.
|
US
|
Ian Finder Senior Program Manager, Microsoft
|
On Demand
|
by Contributed | Oct 1, 2020 | Azure, Technology, Uncategorized
This article is contributed. See the original author and article here.
Given the richness and potential sensitivity of data stored in security solutions such as Azure Sentinel they can become important targets for external threat actors who gain access to the environment as well as malicious insiders and therefore should be monitored for specific threats. To assist in this a new feature was recently added to Log Analytics (the technology the underpins Azure Sentinel’s log storage and query capabilities), that allows you to capture an audit log of all queries run against the workspace. In this blog we will look at how we can use this new audit feature to monitor and hunt in these audit logs to find potentially malicious activity.
My colleague Sarah Young has shared a great blog covering some aspects of the audit log and how it can be combined with Azure Activity data to give you a wider perspective on user activity. In this blog I am going to take it a step further and deep dive into the details of the logs and look at some hunting and detection queries for this log source that we recently released.
Details of how to set up this audit logging can be found in the audit log documentation. For this blog we are assuming you are writing the audit logs into an Azure Sentinel workspace. Whilst it is possible to write the logs to other sources such as Azure Storage it is recommended for monitoring that you store in an Azure Sentinel Workspace so that you can leverage its capabilities to support hunting and detections.
Anatomy of the Audit Log
Before we start hunting in the logs we need to understand the structure of it and where to find the most valuable data. From a security perspective there are a few fields of particular interest which the table below details. Once you start collecting these audit logs you should spend some time to ensure that you fully understand what they contain.
Column Name
|
Details
|
Time Generated
|
This is the UTC time that the query was submitted.
|
AADEmail
|
This is the email/UPN of the user who submitted the query.
|
RequestClientApp
|
This is the name of the client type used to run the query, for queries made via the Azure Sentinel UI it will be populated with “ASI_Portal” – this is broadly analogous to a UserAgent.
|
QueryText
|
This is as string of the query text that is being executed. It often includes elements not present in the UI, for example you may see “set query_take_max_records=10001;set truncationmaxsize=67108864;” at the start of a query, this is automatically added by the client and was not necessarily entered by the user themselves.
|
RequestContext
|
This is the Azure resource the query was run against. This field is useful if you have the audit log from multiple workspaces aggregated in one workspace as this helps you identify the target workspace for the query. If the query covers multiple workspaces there will be multiple values here.
|
ResponseCode
|
The is the HTTP response code from submitting the query i.e. 200 indicates the query executed without issue.
|
ResponseRowCount
|
The number of result rows the query returned.
|
ResponseDurationMs
|
How long the query took to execute and return a response.
|
Hunting and Detection Opportunities
ATT&CK T1530, T1213, T1020
One of the most obvious and easy detection opportunities with these audit logs is to look for specific key words in the queries being run. Given that security logs often contain an abundance of personal detail a malicious user may want to search the data for specific user activity. We can easily identify this by looking for queries that contains unique identifiers for those users. Below is an example of a query that looks for queries that contains the email addresses of specified VIP users. You could also modify this to include searches for display names or other identifiers. Whilst a security analyst may have legitimate reason to search based on a specific user identifier such as this it would not be expected to be a regular search unless analysts were given specific tasking.
let vips = dynamic(['vip1@email.com','vip2@email.com']);
let timeframe = 1d;
LAQueryLogs
| where TimeGenerated > ago(timeframe)
| where QueryText has_any (vips)
| project TimeGenerated, AADEmail, RequestClientApp, QueryText, ResponseRowCount, RequestTarget
ATT&CK T1530, T1213
In a similar manner as the query above we can also look for users querying for secrets within logs. It is not uncommon for passwords and other secrets to leak into logs and a malicious actor could easily use KQL to search for these. There have been several high profile incidents where plain text credential’s appeared in logs, such as the incident Twitter reported in 2018, and attackers are likely to take advantage of access to a SIEM solution such as Azure Sentinel to check for this. For more on hunting for credentials in datasets please take a look at this RSA blog.
Given the numerous cases where a user might legitimately search of strings such ‘password’ you will need to tailor the keyword and exclusion lists for your environment.
// Extend this list with items to search for
let keywords = dynamic(["password", "pwd", "creds", "credentials", "secret"]);
// To exclude key phrases or tables to exclude add to these lists
let table_exclusions = dynamic(["AuditLogs","SigninLogs", "LAQueryLogs", "SecurityEvent"]);
let keyword_exclusion = dynamic(["reset user password", "change user password"]);
let timeframe = 7d;
LAQueryLogs
| where TimeGenerated > ago(timeframe)
| where RequestClientApp != 'Sentinel-General'
| extend querytext_lower = tolower(QueryText)
| where querytext_lower has_any(keywords)
| project TimeGenerated, AADEmail, QueryText, RequestClientApp, RequestTarget, ResponseCode, ResponseRowCount, ResponseDurationMs, CorrelationId
| extend timestamp = TimeGenerated, AccountCustomEntity = AADEmail
| join kind=leftanti ( LAQueryLogs
| where TimeGenerated > ago(timeframe)
| where RequestClientApp != 'Sentinel-General'
| extend querytext_lower = tolower(QueryText)
| where QueryText has_any(table_exclusions) or querytext_lower has_any(keyword_exclusion))
on CorrelationId
ATT&CK T1530, T1213
An attacker who compromises an account could leverage that account to access Azure Sentinel/Log Analytics even if that user does not regularly use the service. Therefore we can hunt for situations where we see a user executing queries who has not recently done so. This query is likely to be too false positive prone to be useful as detection logic but makes a useful hunting starting point.
let lookback = 7d;
let timeframe = 1d;
LAQueryLogs
| where TimeGenerated between(startofday(ago(lookback))..startofday(ago(timeframe)))
| summarize by AADEmail
| join kind = rightanti (LAQueryLogs
| where TimeGenerated > ago(timeframe))
on AADEmail
| project TimeGenerated, AADEmail, QueryText, RequestClientApp, RequestTarget
| extend timestamp = TimeGenerated, AccountCustomEntity = AADEmail
ATT&CK T1530, T1213
As with new user query we can also hunt for new Service Principals running queries. It is important to cover this perspective as well as Service Principals can also be given access to run queries. Whilst the number of Service Principals running queries is likely to be more static than with users this query is still likely to be false positive prone and therefore is better suited as a hunting query.
let lookback = 7d;
let timeframe = 1d;
LAQueryLogs
| where TimeGenerated between (ago(lookback)..ago(timeframe))
| where ResponseCode == 200 and RequestClientApp != "AppAnalytics" and AADEmail !contains "@"
| distinct AADClientId
| join kind=rightanti(
LAQueryLogs
| where TimeGenerated > ago(timeframe)
| where ResponseCode == 200 and RequestClientApp != "AppAnalytics" and AADEmail !contains "@"
)
on AADClientId
| extend timestamp = TimeGenerated, AccountCustomEntity = AADEmail
ATT&CK T1530, T1213, T1020
Often when an account is compromised by an external attacker there are indicators that the account is not being controlled by a legitimate user, one of these indicators can be when the account uses a technology not commonly associated with regular users. We can look for this in the audit log by monitoring for queries executed by a client type we have not previously seen. This query can also be combined with some of the anomaly detection queries detailed later in order to create a more refined detection or hunting query.
let lookback = 7d;
let timeframe = 1d;
LAQueryLogs
| where TimeGenerated between (ago(lookback)..ago(timeframe))
| where ResponseCode == 200
| join kind= rightanti(
LAQueryLogs
| where TimeGenerated > ago(timeframe)
)
on RequestClientApp
| extend timestamp = TimeGenerated, AccountCustomEntity = AADEmail
ATT&CK T1030
A malicious actor with access to a data source may look to exfiltrate date from the source for persistent offline access. During the 2014 breach of Anthem attackers searched across datasets to find material of interest and then extracted these in bulk queries . We can monitor this activity such as this by looking for multiple large queries made by a single user. Most legitimate users are unlikely to run multiple queries that return many thousands for rows as data that size is unmanageable from an analysis perspective.
let UI_apps = dynamic(['ASI_Portal','AzureMonitorLogsConnector','AppAnalytics']);
let threshold = 3;
let timeframe = 1d;
LAQueryLogs
| where TimeGenerated > ago(timeframe)
| where (ResponseRowCount == 10001 and RequestClientApp in(UI_apps)) or (ResponseRowCount > 10001 and RequestClientApp !in(UI_apps))
| summarize count() by AADEmail
| where count_ > threshold
| join kind=rightsemi (
LAQueryLogs
| where TimeGenerated > ago(timeframe)
| where (ResponseRowCount == 10001 and RequestClientApp in(UI_apps)) or (ResponseRowCount > 10001 and RequestClientApp !in(UI_apps)))
on AADEmail
| extend timestamp = TimeGenerated, AccountCustomEntity = AADEmail
ATT&CK T1030
As well as just looking for large query results, we can use KQL’ timeseries capabilities to look for anomalies in the volume of data being returned by a user. Whilst this may generate some false positives for users who only use the service occasionally it is an effective tool for identifying suspicious changes in regular user’s activity.
let lookback = 7d;
let threshold = 0;
LAQueryLogs
| make-series rows = sum(ResponseRowCount) on TimeGenerated in range(startofday(ago(lookback)), now(), 1h)
| extend (anomalies, score, baseline) = series_decompose_anomalies(rows,3, -1, 'linefit')
| mv-expand anomalies to typeof(int), score to typeof(double), TimeGenerated to typeof(datetime)
| where anomalies > threshold
| sort by score desc
| join kind=rightsemi (
LAQueryLogs
| summarize make_set(QueryText) by AADEmail, RequestTarget, TimeGenerated = bin(TimeGenerated, 1h))
on TimeGenerated
| project TimeGenerated, AADEmail, RequestTarget, set_QueryText
| extend timestamp = TimeGenerated, AccountCustomEntity = AADEmail
ATT&CK T1530, T1213, T1020
As well as looking for anomalies in data volume we can also look for anomalies in the number of different workspaces that a user is querying. A user who regularly runs queries against a single workspace who then suddenly starts querying multiple workspaces is a potential indicators of malicious activity.
let lookback = 30d;
let timeframe = 1d;
let threshold = 0;
LAQueryLogs
| where TimeGenerated between (ago(lookback)..ago(timeframe))
| mv-expand(RequestContext)
| extend RequestContextExtended = split(RequestTarget, "/")
| extend Subscription = tostring(RequestContextExtended[2]), ResourceGroups = tostring(RequestContextExtended[4]), Workspace = tostring(RequestContextExtended[8])
| summarize count(), HistWorkspaceCount=dcount(Workspace) by AADEmail
| join (
LAQueryLogs
| where TimeGenerated > ago(timeframe)
| mv-expand(RequestContext)
| extend RequestContextExtended = split(RequestTarget, "/")
| extend Subscription = tostring(RequestContextExtended[2]), ResourceGroups = tostring(RequestContextExtended[4]), Workspace = tostring(RequestContextExtended[8])
| summarize make_set(Workspace), count(), CurrWorkspaceCount=dcount(Workspace) by AADEmail
) on AADEmail
| where CurrWorkspaceCount > HistWorkspaceCount
// Uncomment follow rows to see queries made by these users
//| join (
//LAQueryLogs
//| where TimeGenerated > ago(timeframe))
//on AADEmail
//| extend timestamp = TimeGenerated, AccountCustomEntity = AADEmail
ATT&CK T1530, T1213, T1020
As well as looking for timeseries based anomalies in data volume we can also look for situations where a specific users total returned rows of data for a day increases significantly from the last seven days. Whilst this may produce false positives in occasional users it can be a useful hunting query when looking at users who regularly run queries but who suddenly exhibit suspicious behavior.
let threshold = 10;
let lookback = 7d;
let timeframe = 1d;
let baseline = 10000;
let diff = 5;
let anomolous_users = (
LAQueryLogs
| where TimeGenerated between(startofday(ago(lookback))..startofday(ago(timeframe)))
| summarize score=sum(ResponseRowCount) by AADEmail
| join kind = fullouter (LAQueryLogs
| where TimeGenerated > startofday(ago(timeframe))
| summarize score_now=sum(ResponseRowCount) by AADEmail)
on AADEmail
| extend hist_score = iif((score/29)*threshold > baseline, (score/29)*threshold, baseline)
| where isnotempty(score)
| where score_now > hist_score*diff
| project AADEmail);
LAQueryLogs
| where TimeGenerated > ago(timeframe)
| where AADEmail in(anomolous_users)
| extend timestamp = TimeGenerated, AccountCustomEntity = AADEmail
// Comment out the line below to see the queries run by users.
| summarize total_rows = sum(ResponseRowCount), NoQueries = count(), AvgQuerySize = sum(ResponseRowCount)/count() by AADEmail
ATT&CK T1020
Another indicator of suspicious activity is the repeated failure of queries. Regular users are unlikely to have many queries that fail repeatedly. Instead this is likely be caused by misconfigured automated queries. Legitimate services are likely to catch and remediate such issues quickly so searching for repeated failures can help to identify malicious services.
let lookback = 7d;
let timeframe = 1h;
let threshold = 10;
LAQueryLogs
| where TimeGenerated > ago(lookback)
| where ResponseCode != 200
| summarize count() by AADEmail, bin(TimeGenerated, timeframe)
| where count_ > threshold
| join kind=rightsemi (
LAQueryLogs
| where TimeGenerated > ago(lookback)
| summarize make_set(QueryText) by AADEmail, bin(TimeGenerated, timeframe))
on AADEmail, TimeGenerated
| extend timestamp = TimeGenerated, AccountCustomEntity = AADEmail
All of the queries detailed here are available via the Azure Sentinel GitHub, in addition we are continuing our work to look at these audit logs and we hope to publish additional material on how to use them to make you queries, and team operations more efficient in the near future.
Thanks to Ashwin Patil, Christopher Glyer and Shain Wray for thier input, and ideas on this topic.
by Contributed | Oct 1, 2020 | Azure, Technology, Uncategorized
This article is contributed. See the original author and article here.
Azure Security Center assesses your environment and enables you to understand the status of your resources, to help you improve the Security Posture. The heart of the Security Center lies in its recommendations. Security recommendations help you to reduce the attack surface across your workloads. One important recommendation that Security Center has is to identify if your computers have an endpoint protection solution installed.
Azure Security Center monitors the status of antimalware protection and reports this under the ‘Enable Endpoint protection’ security control when it identifies the Antimalware solution is not installed or unhealthy. Security Center highlights issues, such as detected threats and insufficient protection, which can make your virtual machines vulnerable to antimalware threats. This is a very important recommendation because malware cannot only be downloaded or installed by threat actors, but also inadvertently by legitimate users who are supposed to access your machines.
Azure Security Center covers a variety of Antimalware vendors today. You can find the list of supported versions in this article. However, in a scenario where you’re using an antimalware protection that is not in the Azure Security Center supported list but you still want to have visibility over the status of this antimalware using Security Center dashboard, how would you tackle this scenario? In this blog, I would like to walk you through a Github artifact that will help you monitor any antimalware you may be using even if it is not in the Security Center supported list.
The Github artifact will deploy a Guest Configuration policy to monitor antimalware in your machines. For context, Azure Guest Configuration Policy can audit settings inside a machine, both for machines running in Azure and Arc Connected Machines, the validation is performed by the Guest Configuration extension and client. Before we deploy this artifact, let’s understand the resources that’s making it all possible.
Expected contents of this Custom Guest Configuration artifact
The completed package is used by Guest Configuration to create the Azure Policy definitions. The package consists of:
– Modules folder
- AzureGuestConfigurationPolicy module
- DscResources module
- (Windows) DSC resource modules required by the MOF
The DSC module in the artifact, is checking CIM Instance (Get-CimInstance) in the SecurityCenter namespace to check for any antivirus product installed in the Windows VM and the query returns the list of antimalware installed in the machine (an example is as shown in Image 1).
Image 1: Get-CimInstance output
In my example here, the VM is running Windows Defender as well as Avast Antivirus. Azure Security Center can discover Windows Defender however, Avast Antivirus discovery is not supported.
The DSC is then trying to identify if there is a process running that executable on the VM, which evaluates the Antivirus is not just installed but it’s running and healthy in a given VM.
The DSC is using Get-Process that will find the process running in the machine (an example is as shown in Image 2). If there’s no instance of a process running the Antivirus executable found, the validation will stop and returns a status of Stopped providing you a clear description.

Image 2: Get-Process output
In summary, If the antimalware is not found in the CIMInstance list, then the DSC returns Absent. If it is found, but doesn’t identify the process running, it will return:
Ensure = “Present”
Status = “Stopped”
If the process is found, then:
Ensure = “Present”
Status = “Running”
For an overview of DSC concepts and terminology, see PowerShell DSC Overview.
Under AzureGuestConfigurationPolicy folder, you’ll find a module (AzureGuestPolicyHelper.psm1) that will help you create policy package, upload it directly to your Azure subscription and will configure everything for you. Let’s take a step back and review the resources in here.
When auditing Windows, Guest Configuration uses a Desired State Configuration (DSC) resource module to create the configuration file. The DSC configuration defines the condition that the machine should be in. If the evaluation of the configuration fails, the policy effect auditIfNotExists is triggered and the machine is considered non-compliant.
In our example, under the Configuration folder you’ll notice a bogus configuration which is needed for Azure Guest Configuration policy during execution. So here, the configuration that will be used for the policy. In our scenario, we want to monitor Antivirus making sure the antivirus is installed and the agent is running. Find the sample below for reference,
Configuration MonitorAntivirus
{
Import-DscResource -ModuleName EndPointProtectionDSC
Node MonitorAntivirus
{
EPAntivirusStatus AV
{
AntivirusName = “Windows Defender”
Status = “Running”
Ensure = “Present”
}
}
}
cd $env:Temp
MonitorAntivirus
NOTE: Though I’m specifying Windows Defender as the AntivirusName in the configuration file, it could really be anything. You could monitor for any Antivirus software you might be running, during the compile time, which takes me to my next section – Parameters file.
The script is using parameters file (sample below for reference) which basically tells the Azure Guest Configuration policy to monitor for that specific Antivirus software that you specify during the assignment.
Remember, you can use wildcard entry too. For eg., if you want to monitor for ‘Avast Antivirus’ and you’re not sure what is the complete name of the Antivirus Windows is referring it as, you can simply type in as Avast*
@(
@{
Name = ‘AntivirusName’
DisplayName = ‘Antivirus Name’
Description = “Name of the Antivirus Software to monitor.”
ResourceType = “EPAntivirusStatus”
ResourceId = ‘AV’
ResourcePropertyName = “AntivirusName”
DefaultValue = ‘Windows Defender’
#AllowedValues = @(‘Avast’,’Windows Defender’,’CrowdStrike’,’Sentinel One’)
}
)
This entire configuration is compiled into a .MOF file which will eventually be stored in the Azure blob storage account that you specify during the execution.
Make sure to read about how Azure Policy’s Guest Configuration works that will help you understand this script in detail.
Execution of this Custom Guest Configuration policy:
Pre-requisites:
- Make sure to download the entire artifact from the Github repository and save the unzipped folder to your local machine under ‘C:Program FilesWindowsPowerShellModules’ before the execution.
- To audit settings inside a machine, the Guest Configuration extension and a managed identity is required to audit Azure virtual machines. To deploy the extension at scale, make sure to choose appropriate scope and assign the policy initiative ‘Deploy prerequisites to enable Guest Configuration policies on virtual machines’ under Azure Policies as shown in the image (Image 3) below.
Image 3: Deploy Prerequisites to enable Guest Configuration Policies in VMs
Refer to this article, to understand Scope in Azure Policy.
Image 4: Policies under Guest Configuration Policy Initiative
To understand the Effect Type of the policies, please refer to this article
Once you’ve assigned the Guest Configuration policy to a specific Scope, you can monitor the Compliance of the policy from the ‘Compliance’ section under ‘Azure Policy’. As shown in the below Image 5.
Image 5: Guest Configuration Policy Initiative showing Compliance
Once you have taken care of the pre-requisites, proceed with the execution of the policy.
Execution:
- Open the PowerShell as an administrator and run the command ‘New-EPDSCAzureGuestConfigurationPolicyPackage’ which will create policy package for you in your Azure Subscription.
- You’ll first be prompted to enter a Resource Group Name, Resource Group Location, Storage Container Name, Storage account name and Storage SKU name
- You’ll then be prompted to login to your Azure Subscription
Refer to this article to learn about Storing Guest Configuration artifacts.
- Once connected to your subscription, the script will compile the DSC configuration to a MOF file and will publish the Configuration package to the storage account (you defined in Step 2) in the blob storage (as shown in the below image 6)
- This eventually generates a Guest Configuration policy and publishes the Initiative under Definitions of the Azure Policies as a Guest configuration policy.
- Under the Policies tab, you’ll notice all the individual policy definitions in the initiative that contribute to this control, as shown in image 7 and Image 8.
Policy Execution
Image 6: Configuration file that’s uploaded to Storage blob Container
Image 7: Initiative to monitor Antivirus
Image 8: Policies under the Initiative
Now it’s time to assign this Initiative to the scope you desire to target. The term scope refers to all the resources, resource groups, subscriptions, or management groups that the definition is assigned to.
Please refer this article, to learn more about the assignment and for steps to assign it via PowerShell and Azure CLI.
While you’re assigning this Initiative to a Scope, you could modify the Assignment name if required as shown in the gif below.
Policy Assignment
Once you’ve assigned the custom policy, review the Compliance state of the policies by navigating to ‘Compliance’ under Azure Policy and selecting the Initiative. The Resource compliance tab provides a granular view of each resource that’s evaluated by a member policy of the currently viewed control.
Checking Compliance
In my example, I’m monitoring to gather details of VMs which do not have installed and not running. I’ve two resources (epptest (VM without Avast Installed) and vmclient (VM with Avast Installed)) in the selected scope. The script is identifying the VM that do not have Avast installed and raising the non-compliance of the resource with the help of ‘Audit policy’.
Assign the Custom Initiative to Azure Security Center
You can add the custom Initiative to Security Center to receive recommendations if your environment doesn’t follow the policy you created. As we learnt above, Security Center automatically runs continuous scans to analyze the security state of your Azure resources. When Security Center identifies potential security vulnerabilities, it creates recommendations that guide you through the process of configuring the needed security controls. Security Center updates the Endpoint Protection issue recommendations within 8 hours.
To assign this Custom Initiative, navigate to Security Policy under Security center and choose the desired subscription or Management Group. Click on ‘Add a Custom Initiative’ under Your Custom Initiatives that should take you to a screen as below (Image 9), find the Initiative created above and click on ‘add’
Image 9: Add Custom Initiative
Your new Initiative takes effect and you can see the impact in two ways:
- From the Security Center sidebar, under Policy & Compliance, select Regulatory compliance. The compliance dashboard opens to show your new custom initiative alongside the built-in initiatives.
- You’ll begin to receive recommendations if your environment doesn’t follow the policy you’ve defined.
To see the resulting recommendations for your policy, click Recommendations from the sidebar to open the recommendations page. The recommendations will appear with a “Custom recommendations” label as shown in Image 10
Image 10: Custom Recommendations
NOTE: Make sure to disable the Endpoint protection policy (in the built-in ASC initiative) that generates missing Endpoint protection recommendation inorder to prevent it appearing again and to avoid it to be counted to the overall Secure score.
The disable policy changes can take up to 12 hours to take effect.
Refer to this article to disable a recommendation to appear by disabling the specific policy that generates the recommendations.
Enhance custom Recommendations
You can further enhance your custom recommendations similar to the built-in recommendations. The built-in recommendations supplied with Azure Security Center includes details such as severity levels and remediation instructions. If you want to add this type of information to your custom recommendation, follow this article
This GitHub Artifact as well as many other can be found here:
Direct Link to GitHub sample
Azure Security Center GitHub Repo
Additional Resource:
Big shoutout to Microsoft365DSC which made this all possible. Check it out.
Are you an organization using Endpoint protection outside of what ASC supports? Don’t worry anymore, go ahead and deploy this policy to witness the magic.
Reviewer
Special Thanks to @Yuri Diogenes, Principal Program Manager in the CxE ASC Team for reviewing this article.
by Contributed | Oct 1, 2020 | Azure, Technology, Uncategorized
This article is contributed. See the original author and article here.
Microsoft partners like Unravel Data, Tidal Migrations, and NGINX deliver transact-capable offers, which allow you to purchase directly from Azure Marketplace. Learn about these offers below:
 |
Unravel for Azure HDInsight: This monitoring, tuning, and troubleshooting tool from Unravel gives you instant visibility into Microsoft Azure HDInsight clusters with a single click. Unravel provides AI-powered recommendations and automated actions to optimize big data pipelines and applications, maximizing cost savings. Unravel for Azure HDInsight installs Unravel on an edge node in your cluster. Try it free for 14 days.
Unravel for Azure Databricks and Azure HDInsight: Unravel’s monitoring, tuning, and troubleshooting tool for Spark on Microsoft Azure Databricks and HDInsight provides granular chargeback and cost optimization for big data workloads. Evaluate your migration from on-premises Hadoop to Azure, optimize your cluster resources, provide essential context to DataOps teams, and maximize Spark performance and reliability in the cloud.
|
 |
Tidal Migrations Platform: The Tidal Migrations platform provides your team with a simple, fast, and cost-effective cloud migration management solution. Its efficient tools enable you to gather and organize information in an application-centric way, with source code analysis and PDF cloud application assessment reports. Share dashboards and collaborate as you discover, assess, plan, and execute your migration to Microsoft Azure.
Tidal Migrations -Premium Insights for Database: This add-on to your Tidal Migrations subscription enables you to quickly analyze your databases, identifying roadblocks to cloud migrations based on more than 100 unique characteristics. Databases are analyzed based on their metadata, looking at specific schema objects and permissions, as well as any proprietary features that won’t be available in the target platforms.
Tidal Migrations -Premium Insights for Source Code: Use this add-on to your Tidal Migrations subscription to provide insights about the applications you plan to refactor or replatform to Microsoft Azure. Scan your application source code, identifying roadblocks to cloud migrations based on 140+ patterns. Detect the frameworks in use (Struts, Rails, jQuery, etc.) as well as vulnerabilities in your code today.
|
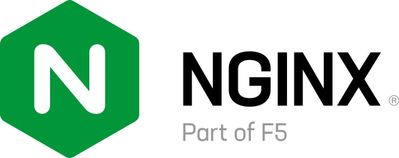 |
NGINX Plus: Deliver applications with performance, reliability, security, and scale with NGINX Plus on Microsoft Azure. NGINX Plus is lightweight yet serves as an all-in-one load balancer, reverse proxy, API gateway, and content cache. Deploy quickly and cost-effectively with NGINX Plus, and benefit from speeds of less than 30 milliseconds.
|
|
by Contributed | Oct 1, 2020 | Azure, Technology, Uncategorized
This article is contributed. See the original author and article here.
Minimizing business downtime during planned maintenance
Introduction
I was preparing a proposal for a customer recently and one of the pain points with their current SAP infrastructure architecture was that they were unable to patch their SAP environment without impacting their business with planned downtime.
Given the business criticality of SAP to this customer, minimizing planned downtime during patching was a key requirement and something that had to be addressed in our SAP on Azure proposal.
An SAP (on Azure) environment is made up of multiple software components that may need to be patched during the lifecycle of the system:
- SAP Application (Enhancement Packages and Support Packages)
- SAP Kernel
- Database
- Operating System
In this blog post I’d like to demonstrate how an SAP on Azure infrastructure deployed following Microsoft’s reference architecture for SAP S/4HANA for Linux VMs on Azure in combination with an SAP native capability called Rolling Kernel Switch (RKS) can enable the SAP Kernel to be patched with minimized business downtime.
It’s important to note that while the detailed steps outlined in this blog post are for Linux, the Rolling Kernel Switch (RKS) will work equally as well in Windows environments on Azure that follow the Microsoft reference architecture for SAP NetWeaver (Windows) for AnyDB on Azure.
Important SAP notes to review in relation to SAP Rolling Kernel Switch (RKS) are:
It is mandatory to read and understand the standard SAP documentation and notes before implementing RKS in your own environment.
Microsoft Reference Architectures for SAP on Azure
The Microsoft reference architecture for SAP S/4HANA for Linux VMs on Azure and reference architecture for SAP NetWeaver (Windows) for AnyDB on Azure show a set of proven practices for running S/4HANA and SAP NetWeaver in a high availability environment that supports disaster recovery on Azure. This architecture is deployed with specific virtual machine (VM) sizes that can be changed to accommodate your organization’s needs.
For the purposes of this blog/demo I’ve built an S/4HANA 1809 on Azure environment that follows the reference architecture with the following exceptions:
The SAP components of the reference architecture for SAP S/4HANA for Linux VMs on Azure that we will be patching without system downtime are outlined in green in the schematic below:

Source: Reference architecture for SAP S/4HANA for Linux VMs on Azure
Component
|
Virtual Hostname
|
Operating System
|
HA Cluster
|
SAP ASCS/ERS Node 1
|
anf-ascs
|
SLES for SAP 12 SP4
|
Y
|
SAP ASCS/ERS Node 2
|
anf-ers
|
SLES for SAP 12 SP4
|
Y
|
SAP Primary Application Server (PAS)
|
sapapp1
|
SLES for SAP 12 SP4
|
n/a
|
SAP Additional Application Server (AAS)
|
sapapp2
|
SLES for SAP 12 SP4
|
n/a
|
The current kernel patch level is 773 patch 101 and this will be patched to level 201 using RKS.
It is important to note that while the detailed steps in this blog are specific to SUSE SLES, the Rolling Kernel Switch (RKS) can be implemented in a similar fashion for SAP NetWeaver on RHEL HA environments on Azure.
Rolling Kernel Switch (RKS)
SAP provides detailed guidance on the RKS process in the SAP NetWeaver 7.4 Administration Guide.
In addition, SAP note 953653 – Rolling Kernel Switch contains important pre-requisites and restrictions. In particular, it should be noted that there is a manual RKS procedure for 7.2x kernel releases. As of 7.4x an automatic RKS procedure is provided by SAP.
It is mandatory to read and understand the standard SAP documentation and notes before implementing RKS in your own environment.
In summary, the rolling kernel switch (RKS) is an automated procedure that enables the SAP kernel in an SAP ABAP system to be exchanged without system downtime (for dual-stack and AS Java scenario limitations please refer to SAP note 953653).
RKS can also be used to make parameter changes while the system is running. Usually, RKS only causes minimal restrictions for users of the system.
In the SAP NetWeaver 7.5 Admin Guide, SAP states that the advantages of the rolling kernel switch are:
- SAP kernel exchange without system downtime (Note: individual SAP application instances are re-started but there is no overall system downtime)
- The procedure is automated
- The procedure can be started and monitored using standard tools in SAP MMC and in the system overview (transaction SM51)
- No or minimal impact on system users
- Static parameters can be changed while the system is running
Note: Parameters that affect the whole system should be checked carefully. Parameters that affect the system landscape (e.g. with ASCS instance or database in their name) cannot be changed with RKS.
Source: Automated Rolling Kernel Switch (RKS) in the SAP NetWeaver 7.5 Admin Guide
The intent of this blog is not to repeat the SAP documentation, rather, the key steps in the RKS process are outlined, in particular any steps that are relevant to the Microsoft reference architecture for SAP S/4HANA for Linux VMs on Azure are called out.
RKS Pre-Checks
Before the RKS process can be executed there are pre-requisite checks that must be carried out beforehand. Some of these are manual and must be performed by the system administrator. Other are automatic checks that are executed by the RKS process itself.
RKS manual Pre-checks
SAP lists the manual checks and preparations as follows:
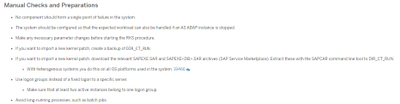
Source: RKS – Manual and Automatic Checks of the System Configuration in the SAP NetWeaver 7.5 Admin Guide
Let’s consider each of these manual checks in turn:
- No component should form a single point of failure in the system
Because we have followed the Microsoft reference architecture for SAP S/4HANA for Linux VMs on Azure (or reference architecture for SAP NetWeaver for AnyDB on Azure) we know that no single SAP component forms a single point of failure in the system:
- SAP Central Services – deployed as a 2 node HA Cluster
- Minimum of 2 SAP Application Servers – To manage logon groups for ABAP application servers, the SMLG transaction is used. It uses the load balancing function within the message server of the Central Services to distribute workload among SAP application servers pool for SAPGUIs and RFC traffic. The application server connection to the highly available Central Services is through the cluster virtual network name. This avoids the need to change the application server profile for Central Services connectivity after a local failover.
- The system should be configured so that the expected workload can also be handled if an AS ABAP instance is stopped
The expected workload on my SAP on Azure demo environment is minimal, however, in a real Production environment this is a key consideration and is why SAP recommends performing RKS activities during periods of low business activity if possible.
- Make any necessary parameter changes before starting the RKS procedure
One of the additional benefits of RKS is that it can be used to implement SAP profile parameter changes without planned business downtime.
Note: Parameters that affect the whole system should be checked carefully. Parameters that affect the system landscape (e.g. with ASCS instance or database in their name) cannot be changed with RKS.
- If you want to import a new kernel patch, create a backup of DIR_CT_RUN
We definitely want to import a new kernel patch so let’s quickly confirm the location of DIR_CT_RUN. I checked using transaction AL11:

Create a backup of DIR_CT_RUN is also easy. For the purposes of this demo I’ve simply created a copy using the o/s command cp as follows:
cp -avr /usr/sap/A4H/SYS/exe/uc/linuxx86_64 /usr/sap/A4H/SYS/exe/uc/linuxx86_64_backup_11192019
- If you want to import a new kernel patch, download the relevant SAPEXE.SAR and SAPEXE<DB>.SAR from SAP ServiceMarketPlace. Extract these with the SAPCAR command line tool to DIR_CT_RUN.
In my case the latest patch level for the complete 773 kernel is 201. Remember to download the DATABASE INDEPENDENT archive AND the DATABASE SPECIFIC archive. In my case SAP HANA.
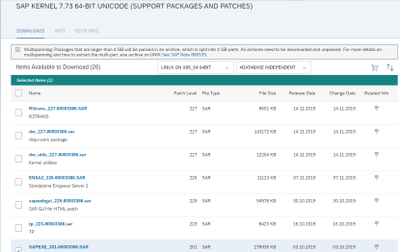
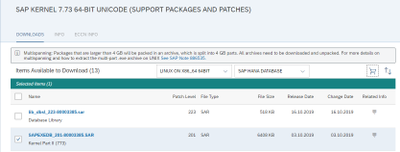
Extract with SAPCAR into DIR_CT_RUN e.g.
cd /sapmnt/A4H/exe/uc/linuxx86_64
SAPCAR -xvf /sapsoftware/SAPKernel773_PatchLevel_201/SAPEXEDB_201-80003385.SAR
SAPCAR -xvf /sapsoftware/SAPKernel773_PatchLevel_201/SAPEXE_201-80003386.SAR
- Use logon groups instead of a fixed logon to a specific server
Most SAP Production environments will be using SAP Logon Groups already but if not it’s always a good practice to do so and they can be configured via transaction SMLG. In my demo case I have two SAP application severs configured in a single Logon Group:
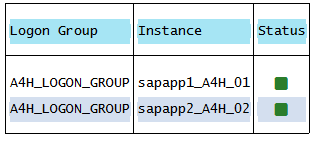
- Avoid long running processes such as batch jobs
In my demo environment this isn’t an issue, however, it very likely will be in real Production system. This is also why SAP recommends performing RKS activities at periods of lower system activity. Each SAP application server will be stopped in turn and if there are still long running batch jobs running on the application server these will be terminated.
RKS Automatic Pre-Checks
The list of RKS automatic checks is extensive and available here
As well as being executed prior to the RKS process itself an Administrator can also execute the automated pre-checks in advance.
To do this, in SAP MMC choose Check Prerequisites from the context menu (right-click) of System Update.
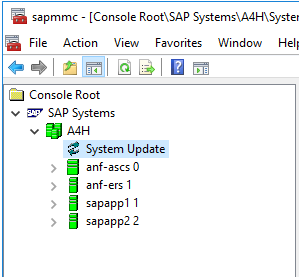
RKS MMC Pre-requisites Check – Error
The first time I executed the pre-requisite checks in my SAP on Azure demo environment I experienced the following error:
FAIL: NIEHOST_UNKNOWN (Invalid argument), <errordetails xmlns=”urn:SAPControl”>NiRawConnect failed in plugin_fopen()</errordetails>
To resolve I uncommented the localhost entry in the hosts file on the VM running the SAM MMC:
# localhost name resolution is handled within DNS itself.
#127.0.0.1 localhost
127.0.0.1 localhost
RKS Pre-requisites Check – Warning
Once the localhosts issue was resolved the next message received was an RKS Warning. RKS had detected – correctly – that my setup was HA using SUSE SLES for SAP Applications 12 SP4.
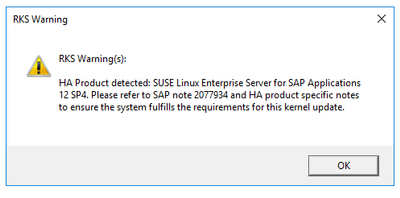
The SAP note in the message and one other referenced within it were relevant to my setup:
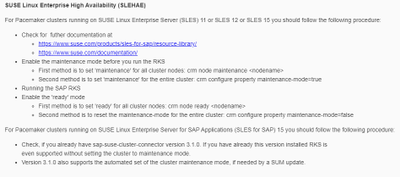
As my HA clusters are based on SLES 12 SP4 I put the ASCS cluster into maintenance mode:

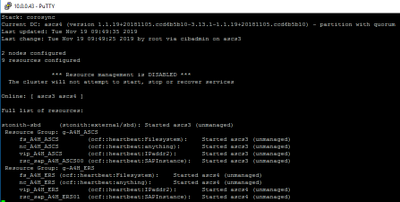
But noted that for Pacemaker clusters running on SUSE Linux Enterprise Server for SAP Applications (SLES for SAP) 15 you should follow the following procedure:
- Check, if you already have sap-suse-cluster-connector version 3.1.0. If you have already this version installed RKS is even supported without setting the cluster to maintenance mode.
RKS Execution
Now that we’ve completed the pre-checks, we’re just about ready to execute the RKS process itself.
SAP provides a detailed description of the RKS Process in the SAP NetWeaver 7.5 Admin Guide
Each component of the SAP system will be stopped in turn:
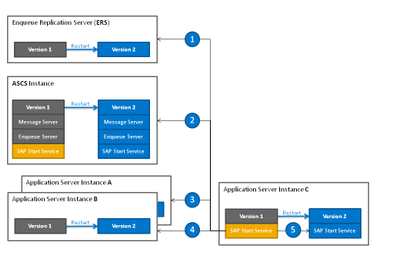
- The enqueue replication server is the first instance restarted.
- The ASCS instance is the second instance restarted.
- Then the application server instances are restarted in the order specified beforehand. In the figure below, instance A is the first application server instance restarted.
- Instance B is the second application server instance restarted, and so on.
- The instance defined as the last one in the order is restarted together with its start service last of all with the new kernel version. The RKS procedure is completed with this final step.
Source: RKS Process in the SAP NetWeaver 7.5 Admin Guide
- Nearly ready to Update System. Before we do let’s just confirm the current kernel patch level of the SAP components that will be updated.
Kernel Patch levels prior to starting the RKS
ERS (dev_enq_replicator):
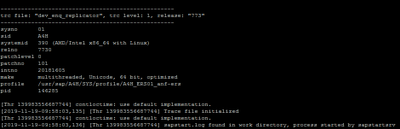
Message Server (dev_ms):
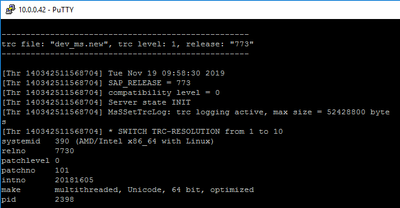
First SAP Application Server:
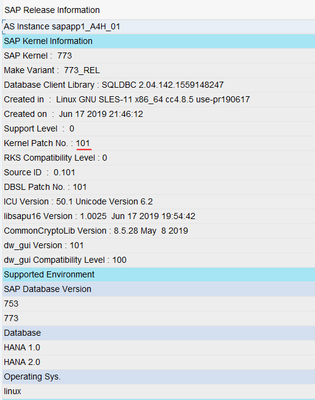
Second SAP Application Server:
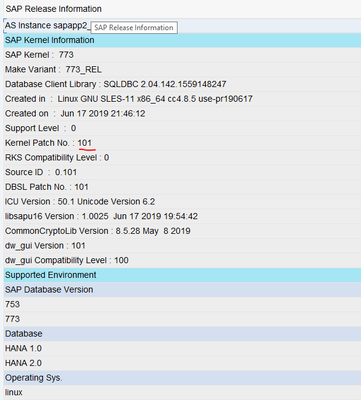
RKS – Update System
To start the RKS Process again I right click on System Update and select Update System from the Context Menu.
Note: You can also start RKS from the command line
In my demo case I reduced the soft shutdown timeout and start wait timeout to lower values than the defaults shown here. In Production environments this should be carefully considered following SAP’s guidance on RKS timeouts.
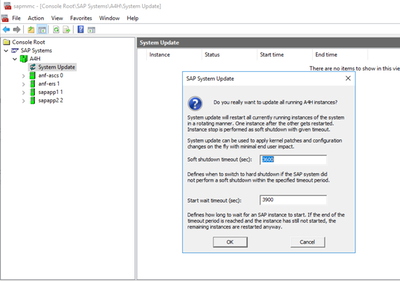
The RKS process re-runs the automated pre-requisite checks we did earlier. As we’ve seen this warning already and read and understood the SAP note 2077934 we can simply click OK.
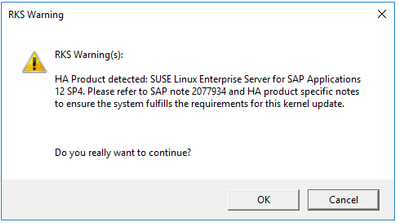
The restart of the ERS and ASCS is very quick (see the RKS log below for detailed timings). Within a few seconds the first SAP application server is shutdown:
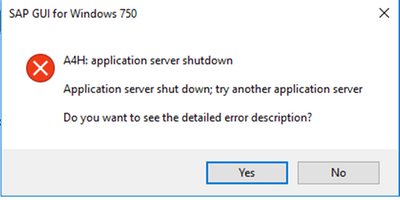
I’m still logged on via the second application server and can see that Kernel update is active in transaction SM51:
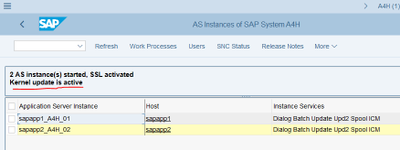
After the first SAP application server is started, the second SAP application server is then shutdown:
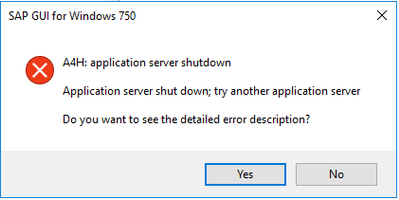
After a few more minutes the process completes successfully.
We can view the complete RKS log via the SAP MMC or directly at the operating system level.
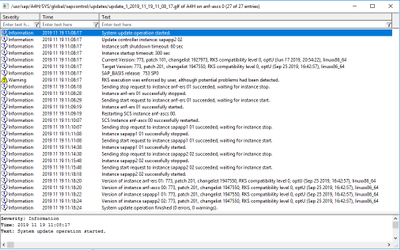
Post RKS Checks
Now that the RKS process has completed we’ll quickly double-check that all the required components have been patched correctly:
The Enqueue replication server looks good – updated to 773 patch 201:
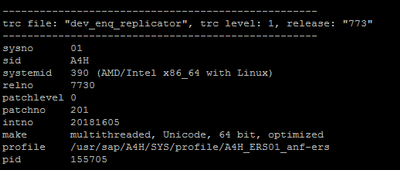
So does the SAP Message Server – updated to 773 patch 201:
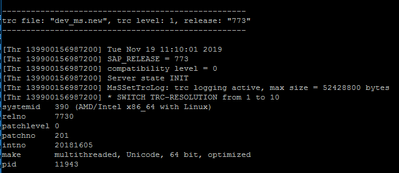
Now let’s check both SAP application servers via transaction SM51 – Release Notes:
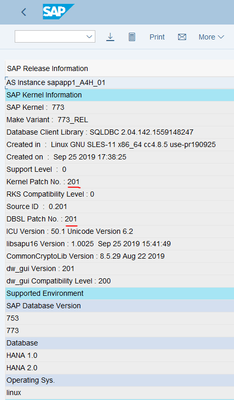
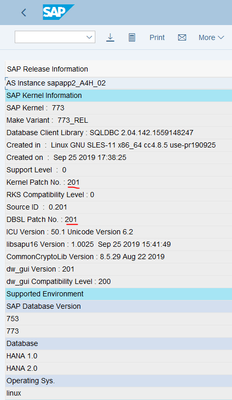
Yup, all good.
We can now take the ASCS Cluster out of maintenance mode:

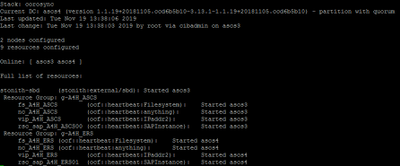
Remembering that per with SLES15 and the sap-suse-cluster-connector version 3.1.0 it will not be necessary to take the cluster in and out of maintenance mode before executing RKS.
Conclusion
An SAP on Azure deployment that follows the Microsoft reference architecture for SAP S/4HANA for Linux VMs on Azure or reference architecture for SAP NetWeaver (Windows) for AnyDB on Azure in combination with the SAP native capability Rolling Kernel Switch is an excellent combination for patching SAP kernels on Azure without system downtime.
Future blog posts will look at downtime minimized patching approaches to other software components of an SAP on Azure environment.
by Contributed | Oct 1, 2020 | Azure, Technology, Uncategorized
This article is contributed. See the original author and article here.
At Microsoft Ignite 2020, we saw a bunch of exciting news announced but we also saw some products renamed and I wanted to create a blog post referencing those changes. So, let’s dive in!
Microsoft 365
Microsoft 365 Changes
The first change I want to cover is Microsoft Threat Protection is now Microsoft 365 Defender. This is really a suite of products, ranging from endpoint protection to safeguarding your email messages and links within them.
Azure Advanced Threat Protection has changed its name to Microsoft Defender for Identity. This product helps you to detect and investigate advanced attacks on prem AND in the cloud.
Microsoft Defender Advanced Threat Protection is now Microsoft Defender for Endpoint, this product helps you detect and remediate advanced attacks on your endpoints. It supports Windows operating systems from Windows 7 to Windows Server 2019, and can also be used on Android, Linux (in preview currently) and MacOS. As well as the name change, we saw support for Microsoft Defender for Endpoint launch support for iOS devices, which is currently in preview. Learn more about the latest endpoint security.
Office 365 Advanced Threat Protection is now Microsoft Defender for Office 365. This product is designed to help protect organisations from malicious attacks through things like scanning email attachments for malware, scanning URLs in emails and documents and checking for unauthorized spoofing. My personal favourite feature of Microsoft Defender for Office 365 is Safe Links, it really does help protect if there are malicious links in emails etc and people click on them.
Azure Defender
Azure Security Centre Standard Edition, is now called Azure Defender for Servers. For Windows this help to monitor and protect your servers with Security Centre presenting the alerts and remediation suggestions. While for Linux it use auditd to collect audit records. With Azure Defender for Servers you get things like Just-in-Time(JIT) VM access, which is a great product for protecting common management ports like RDP or SSH and only having them open when and how you need them.
Azure Security Centre for IoT is now called Azure Defender for IoT. As IoT becomes more and more something we are introducing to our homes and organisations we need to ensure we have an appropriate security posture in place and Azure Defender for IoT can help provide that end to end threat detection and analysis.
Advanced Threat Protection for SQL is now called Azure Defender for SQL. This is Generally Available (GA) for Azure SQL database servers and in preview for SQL servers on machines hosted in Azure, other cloud environments, and even on-premises machines. This product helps to monitor for threats such as SQL injection, brute-force attacks and privilege abuse.
Recent Comments